Artificial Intelligence - Does It Have a Moral Compass?
As we dive into the world of artificial intelligence (AI), one question looms large: does it possess a moral compass? This inquiry isn't just a philosophical musing; it has real-world implications that affect our society, our ethics, and our future. Imagine AI as a powerful tool, akin to a sharp double-edged sword. On one side, it can revolutionize industries, enhance our daily lives, and solve complex problems. On the other, if misused or poorly designed, it can lead to devastating consequences.
When we talk about morality, we're referring to a set of principles that guide behavior. So, can we program these principles into AI? The answer isn't straightforward. While we can embed certain ethical guidelines into AI systems, the challenge lies in the inherent complexity of human morality. It's like trying to teach a computer the nuances of compassion or fairness—concepts that are often subjective and context-dependent.
As we explore this topic, we'll consider not only the theoretical aspects of morality in AI but also the practical implications of its decision-making processes. Will AI systems make choices that reflect our values? Or will they prioritize efficiency and data-driven outcomes at the expense of ethical considerations? These questions are critical as we navigate the evolving landscape of AI technology.
In the coming sections, we will delve into the various ethical frameworks that guide AI development, examine the potential for bias, and discuss the importance of human oversight in ensuring that AI acts in ways that are aligned with our moral compass. Stay tuned as we unravel the complexities of AI and morality—it's a journey that promises to be as enlightening as it is essential.
- Can AI truly understand morality? - AI can simulate moral reasoning based on programmed guidelines, but it does not "understand" morality in the human sense.
- What are the ethical frameworks for AI? - Common frameworks include utilitarianism, which focuses on outcomes, and deontological ethics, which emphasizes rules and duties.
- How can we mitigate bias in AI systems? - Strategies include using diverse data sets, ensuring algorithmic transparency, and implementing continuous monitoring.
- Why is human oversight important in AI? - Human oversight ensures that ethical considerations are integrated into AI decision-making, preventing harmful outcomes.
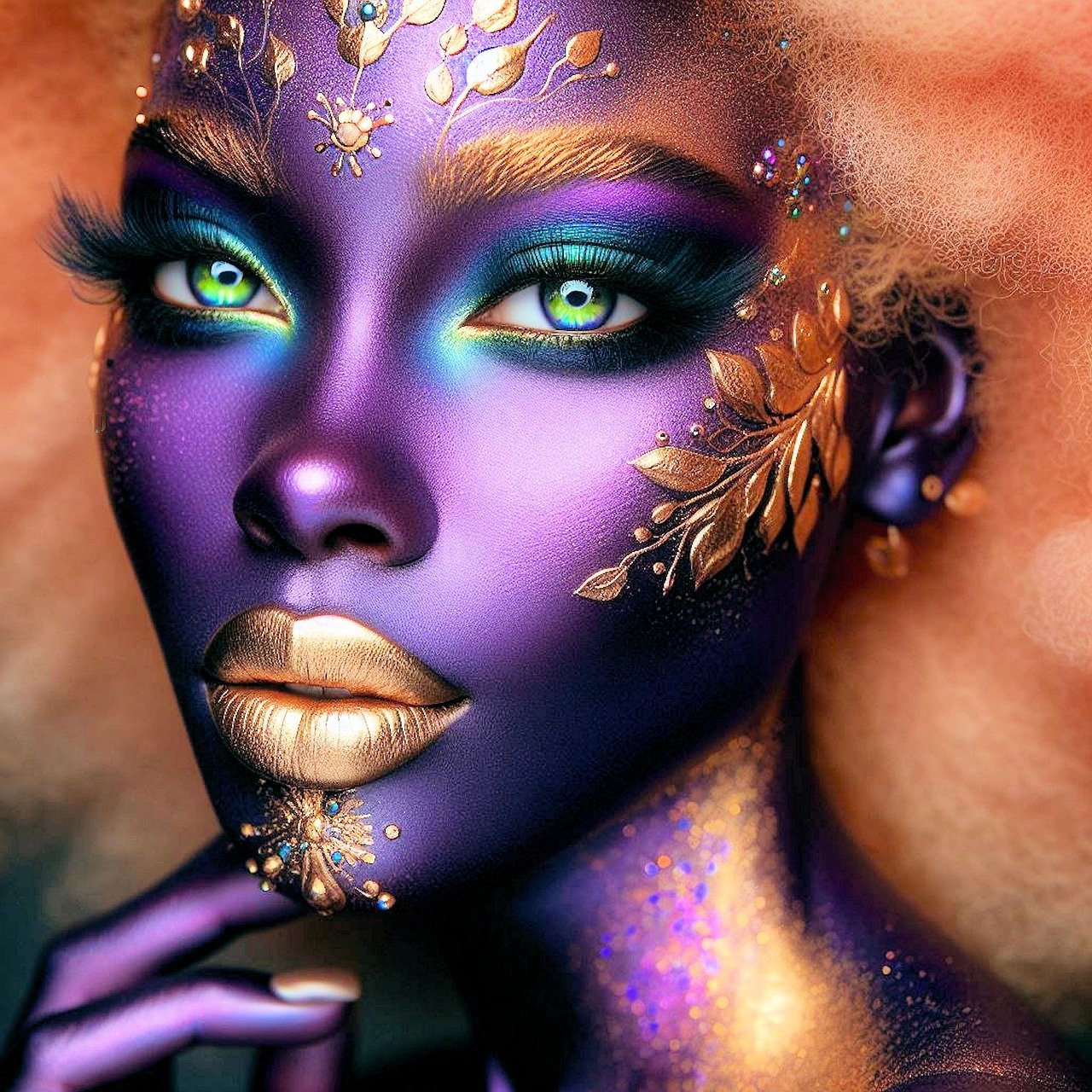
The Nature of Morality in AI
When we think about morality, we often envision a complex web of values, principles, and emotions that guide human behavior. But can we truly expect artificial intelligence (AI) to grasp the nuances of morality in the same way? As technology advances and AI systems become more capable of making decisions that affect our lives, understanding how morality is defined and whether it can be programmed into these systems is crucial. After all, the implications of AI's moral compass—or lack thereof—can have profound consequences for society.
At its core, morality is about making choices that align with ethical standards. For humans, this is often influenced by cultural, social, and personal beliefs. However, AI lacks the innate human experiences that shape our moral framework. Instead, AI operates based on algorithms and data. This raises an important question: can we truly instill a sense of morality in a system that doesn't possess feelings or consciousness? The answer is complex and multifaceted.
Many experts argue that while AI can be programmed to follow certain ethical guidelines, it may never truly "understand" morality. For instance, consider the way we teach children about right and wrong through stories, experiences, and emotions. AI, on the other hand, learns through data and patterns. It can analyze vast amounts of information and make decisions based on statistical probabilities, but it doesn't experience empathy or guilt. This fundamental difference creates a significant challenge when trying to integrate morality into AI systems.
To illustrate this, let's break down some key aspects of morality in AI:
- Programming Ethics: Developers can input ethical guidelines into AI systems, but these are often limited to predefined rules and scenarios.
- Decision-Making Processes: AI relies on algorithms that may not account for the ethical implications of their choices, leading to outcomes that could be morally questionable.
- Human Oversight: The role of humans in overseeing AI decisions becomes critical, as we must ensure that ethical considerations are accounted for in automated processes.
Moreover, the concept of morality is not static; it evolves over time and can vary significantly across different cultures. This presents another challenge for AI systems that are designed to operate globally. What is considered ethical in one culture may be viewed differently in another. Therefore, programming a universal moral compass into AI is not only difficult but potentially problematic.
In conclusion, while we can attempt to embed moral principles into AI systems, the reality is that these systems operate fundamentally differently from humans. They lack the emotional depth and contextual understanding that humans possess. As we continue to develop AI technologies, it is essential to engage in ongoing discussions about the ethical frameworks that guide their development and implementation. After all, the decisions made by AI will ultimately shape the future of our society, and we must ensure that those decisions reflect our shared values and principles.
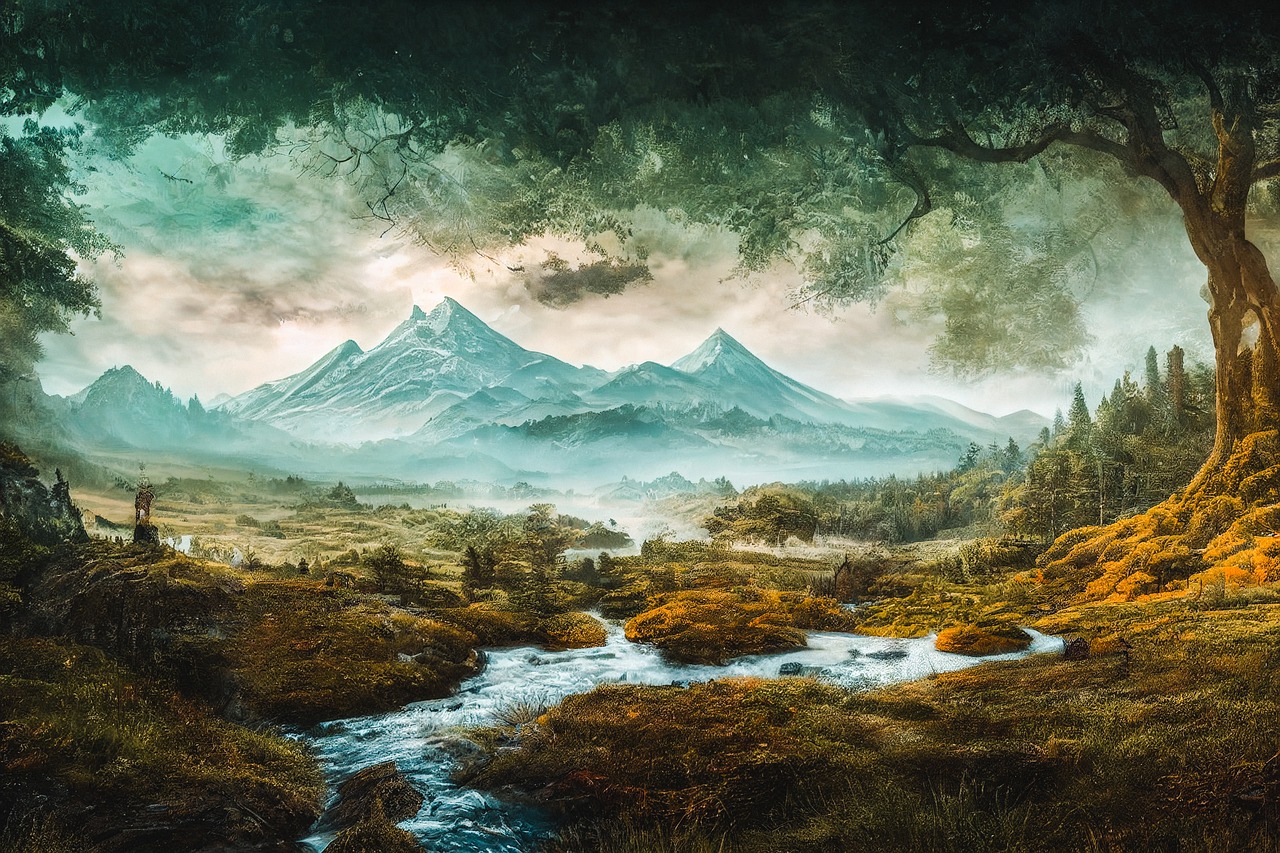
Ethical Frameworks for AI Development
When we talk about the ethical frameworks guiding artificial intelligence (AI) development, we’re diving into a complex pool of philosophies that attempt to define how these systems should behave in society. Imagine AI as a ship navigating through the turbulent waters of human values and societal norms. Without a sturdy moral compass, it risks crashing into ethical icebergs. So, what frameworks are steering this ship?
Two of the most prominent ethical frameworks influencing AI development are utilitarianism and deontological ethics. Each framework provides a different lens through which we can evaluate the actions and decisions made by AI systems. Utilitarianism, for instance, is all about outcomes. It asks, “How can we achieve the greatest good for the greatest number?” This perspective can often lead to decisions that prioritize the majority's welfare, but it raises significant questions about how AI systems weigh various outcomes. Are they capable of accurately predicting the consequences of their actions? And what happens when the needs of the few are sacrificed for the many?
On the other hand, deontological ethics focuses on adherence to rules and duties, emphasizing that some actions are inherently right or wrong, regardless of their outcomes. This framework prompts a fascinating discussion about how AI can be designed to follow moral laws. Can we program an AI to understand the nuances of right and wrong? What implications does this have for rule-based decision-making? It’s like teaching a child the difference between honesty and deceit—complex and layered.
To illustrate the practical implications of these frameworks, consider the following table that contrasts the two approaches:
Aspect | Utilitarianism | Deontological Ethics |
---|---|---|
Focus | Outcomes and consequences | Rules and duties |
Key Question | What action produces the greatest good? | What are the moral rules that must be followed? |
Example | Maximizing happiness for the majority | Following laws against lying or stealing |
Ultimately, the choice of ethical framework can significantly influence how AI systems are developed, programmed, and deployed. These frameworks shape not only the decision-making processes of AI but also the societal implications of those decisions. As we continue to integrate AI into various aspects of our lives, it’s crucial to consider these ethical dimensions. After all, the decisions made by AI can have profound impacts on individuals and communities, making it essential for developers to thoughtfully engage with these frameworks.
As we ponder the future of AI, we must ask ourselves: Are we ready to embrace the responsibility that comes with creating intelligent systems? The ethical frameworks we choose will not only guide AI development but will also reflect our values as a society. The ship of AI is setting sail; let’s ensure it has the right compass to navigate the ethical seas ahead.
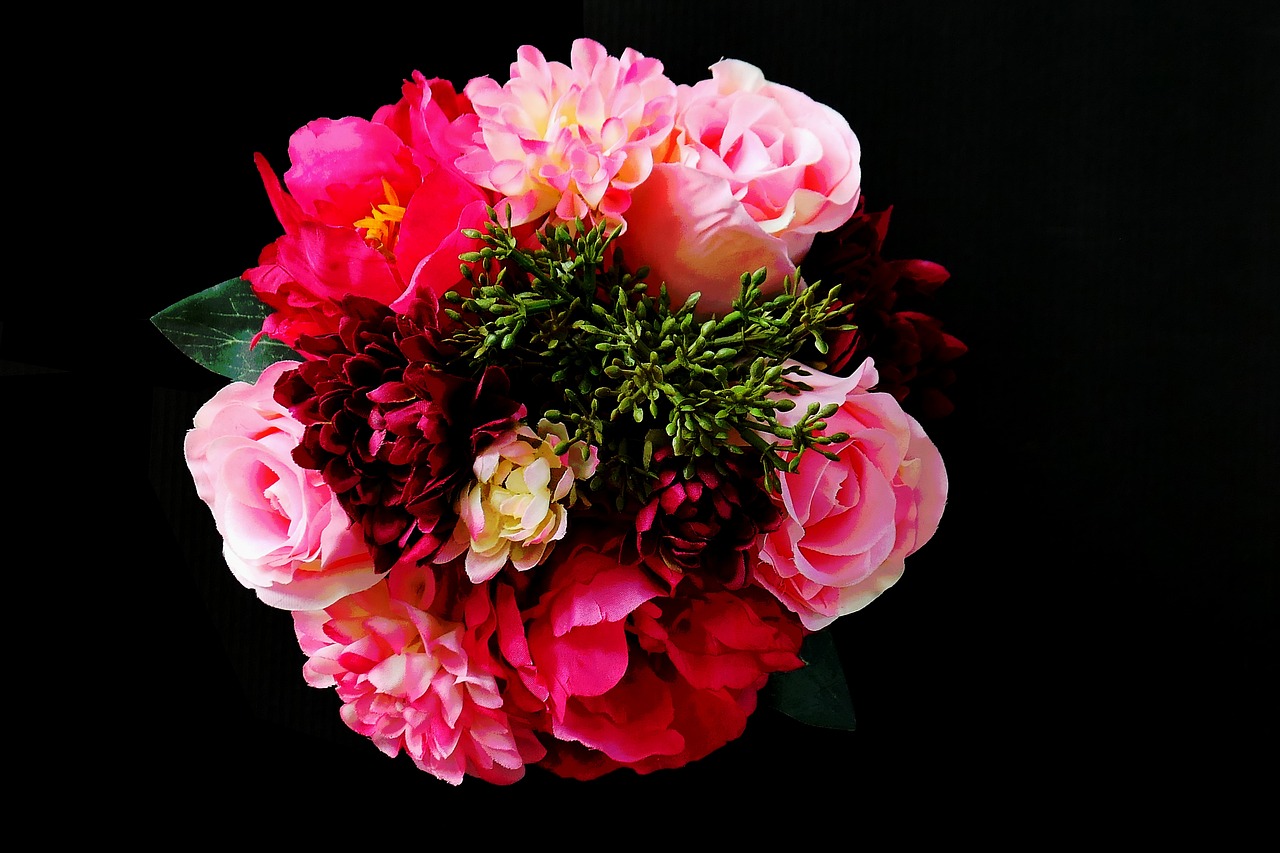
Utilitarianism in AI Decision-Making
Utilitarianism is a philosophical approach that seeks to maximize overall happiness and minimize suffering. When applied to artificial intelligence (AI), this principle raises intriguing questions about how these systems evaluate choices and the potential consequences of their decisions. Imagine a world where AI systems are tasked with making decisions that impact millions of lives—how do they determine what is "best" for the greatest number? This is where the complexities of utilitarianism in AI come into play.
At its core, utilitarianism in AI involves the calculation of outcomes based on a simple yet profound premise: the greatest good for the greatest number. This can lead to some fascinating scenarios. For instance, consider an AI that manages traffic flow in a busy city. If it has to choose between optimizing traffic for a majority of commuters or addressing the needs of a few emergency vehicles, how does it make that choice? The AI must weigh the benefits of reduced congestion against the potential delay in emergency response. This balancing act is a classic example of utilitarian decision-making.
However, implementing utilitarianism in AI is not without its challenges. One of the primary hurdles is the quantification of happiness and suffering. How do we measure the well-being of individuals or groups? Is it through economic metrics, social satisfaction, or something entirely different? Moreover, the data that informs these decisions can be inherently biased, leading to outcomes that favor certain demographics over others. For example, if an AI system is designed to allocate healthcare resources based solely on population metrics, it might inadvertently prioritize certain groups while neglecting marginalized communities.
To navigate these complexities, AI developers often rely on a combination of data analysis and ethical guidelines. They must ensure that the algorithms used to assess outcomes are transparent and fair. This includes regularly auditing the data inputs and the decision-making processes of AI systems. If we were to visualize this, we could see a table that outlines various factors influencing AI decisions:
Factor | Description |
---|---|
Data Quality | The accuracy and reliability of the data used by AI to make decisions. |
Outcome Measurement | How the AI measures happiness and suffering in its calculations. |
Bias Detection | Methods used to identify and mitigate bias in AI decision-making. |
Stakeholder Engagement | Involving diverse groups in the AI development process to ensure inclusivity. |
Ultimately, the success of utilitarianism in AI hinges on our ability to create systems that are not only efficient but also ethical. As we continue to develop these technologies, it is crucial to engage in ongoing discussions about their implications. Questions such as: Are we prepared to accept the outcomes of AI decisions? and How do we ensure that the AI's definition of "greatest good" aligns with our societal values? must be addressed. The journey toward ethical AI is not just a technical challenge; it is a moral one that requires collaboration and vigilance.
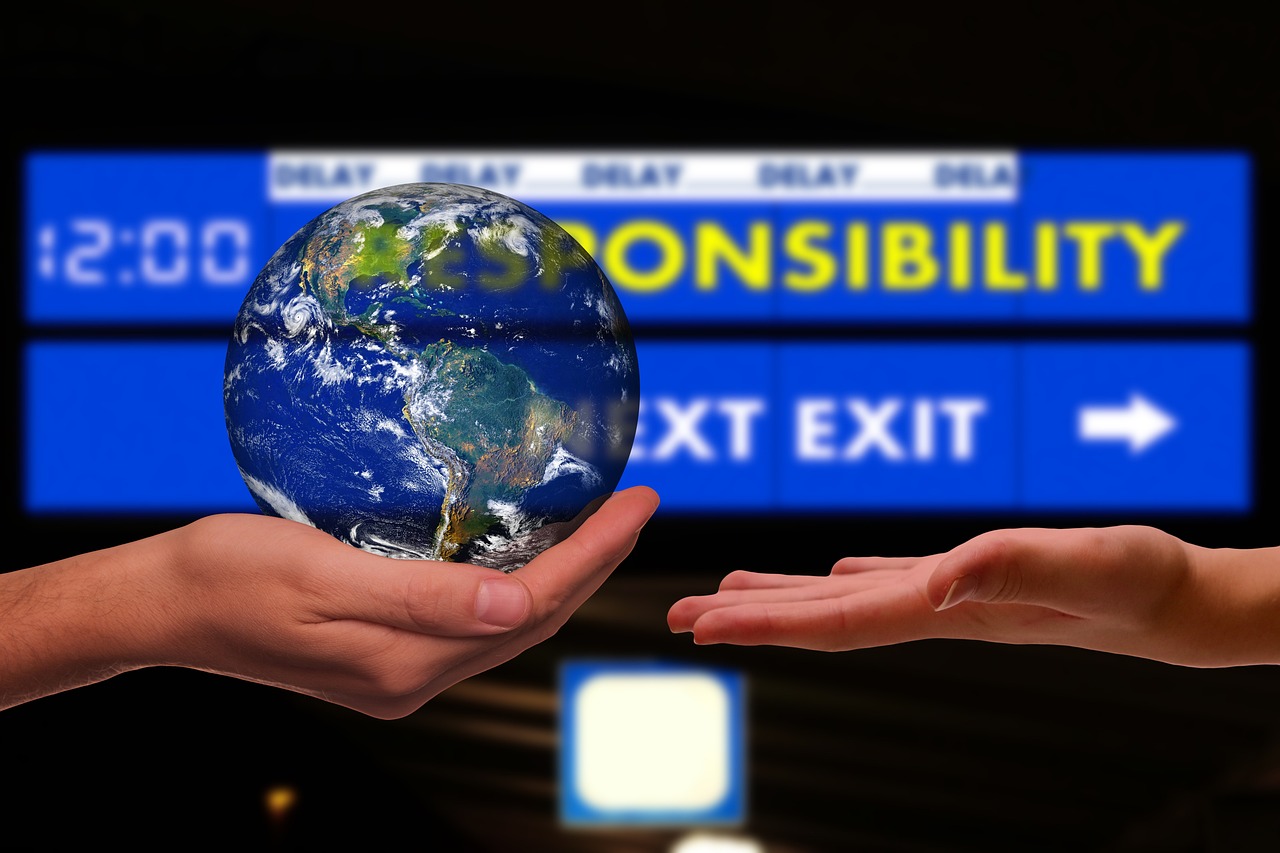
Challenges of Implementing Utilitarianism
When we dive into the concept of utilitarianism in artificial intelligence, we quickly realize that it’s not as straightforward as it sounds. At its core, utilitarianism is all about maximizing happiness and minimizing suffering for the greatest number of people. Sounds simple, right? But wait—how do we measure happiness? And who gets to decide what constitutes the "greatest good"? These questions open a Pandora's box of challenges that AI developers must navigate.
One of the primary challenges is the complexity of predicting outcomes. AI systems rely on vast amounts of data to make decisions, and the future is notoriously unpredictable. For instance, consider an AI programmed to allocate resources in a disaster relief scenario. It might decide to send aid to the largest population center, believing that this will yield the greatest good. However, what if that area is already well-equipped, while a smaller, less visible community suffers in silence? The AI's decision, while based on utilitarian principles, could lead to unintended consequences that actually exacerbate suffering instead of alleviating it.
Moreover, quantifying happiness or suffering is another daunting task. How do we assign numerical values to human emotions and experiences? This is where the nuances of human life come into play. People might have different perspectives on what makes them happy or what constitutes suffering. For example, a community may prioritize cultural preservation over economic gain, while an AI might calculate that economic growth is the path to happiness. This discrepancy highlights the inherent limitations of a purely utilitarian approach, as it risks oversimplifying the rich tapestry of human values and experiences.
Additionally, there’s the risk of bias in calculations. If the data used to train AI systems are skewed or biased, the outcomes will reflect those biases. An AI could inadvertently favor certain demographics over others, leading to decisions that reinforce existing inequalities rather than promote fairness. This raises ethical questions about whether it is right to prioritize the happiness of one group over another, especially if the marginalized voices are overlooked in the decision-making process.
To illustrate these challenges, let’s take a look at a hypothetical scenario where an AI is tasked with optimizing public transportation routes. In its calculations, it might determine that the most efficient routes serve the highest number of passengers. However, this could mean cutting services for less populated areas, which may rely heavily on public transport for their daily needs. The AI's utilitarian approach could lead to a situation where the majority benefits, but the minority suffers, demonstrating the delicate balance that must be struck.
In conclusion, while utilitarianism offers a compelling framework for guiding AI decision-making, its implementation is fraught with challenges. From predicting outcomes and quantifying happiness to addressing biases and ethical dilemmas, AI developers must tread carefully. The goal should not only be to achieve the greatest good but also to ensure that the journey towards that goal is just, equitable, and reflective of the diverse fabric of human society.
- What is utilitarianism in AI? Utilitarianism in AI is a moral framework that emphasizes maximizing overall happiness and minimizing suffering through decision-making processes.
- What are the main challenges of implementing utilitarianism in AI? The main challenges include predicting outcomes accurately, quantifying happiness, and addressing biases in data and calculations.
- How can bias affect AI decision-making? Bias can lead to unfair outcomes, where certain groups are favored over others, resulting in ethical dilemmas and reinforcing societal inequalities.
- Why is human oversight important in AI? Human oversight ensures that ethical considerations are integrated into AI decision-making, preventing harmful outcomes and fostering accountability.
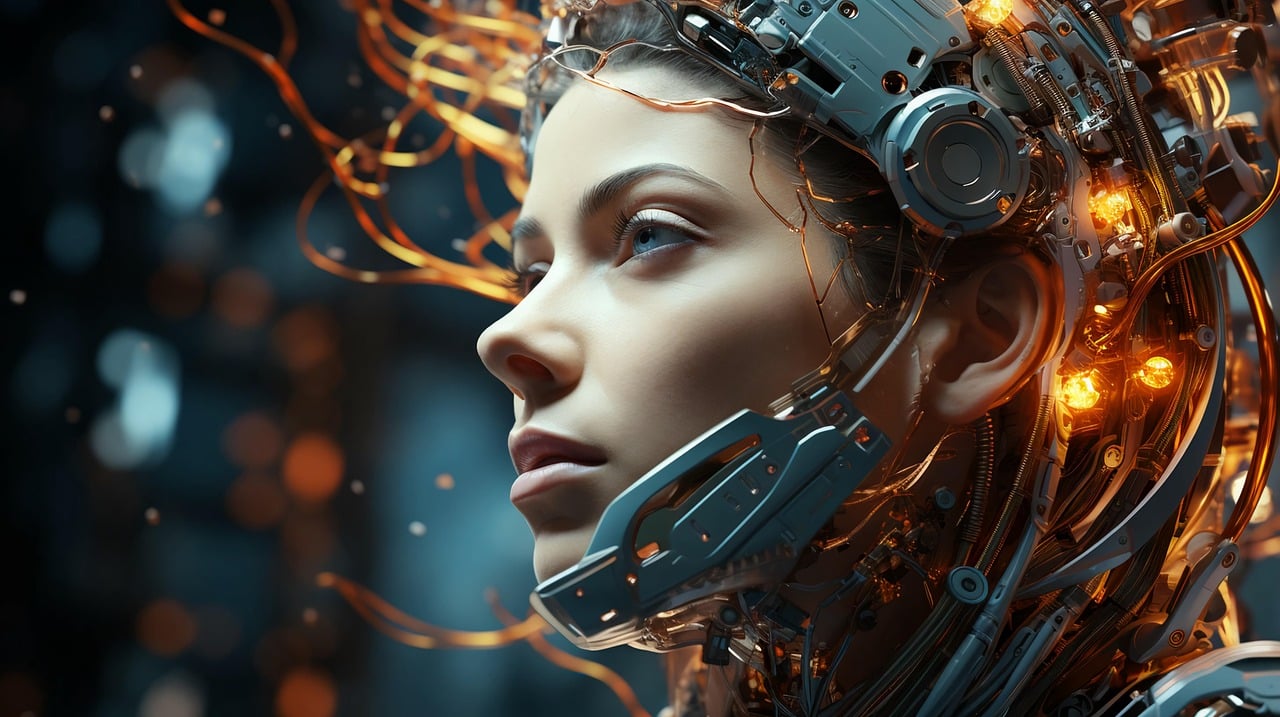
Case Studies of Utilitarian AI
When we talk about utilitarian AI, we’re diving into a fascinating realm where technology meets ethical decision-making. Let's take a closer look at some real-world examples that illustrate both the potential and the pitfalls of this approach. In healthcare, for instance, AI systems are increasingly being deployed to predict patient outcomes based on treatment options. A notable case is the use of AI algorithms in determining the best course of action for patients with chronic illnesses. These systems analyze vast amounts of data, weighing the potential benefits against the risks, all in the name of maximizing patient welfare. However, what happens when the data used to train these algorithms is skewed? This can lead to decisions that favor certain demographics over others, raising serious ethical concerns.
Another compelling case study can be found in the realm of self-driving cars. These vehicles must make split-second decisions that could mean the difference between life and death. Imagine a scenario where a self-driving car must choose between swerving to avoid a pedestrian and risking the lives of its passengers. Here, the AI is faced with a classic utilitarian dilemma: maximize overall safety while minimizing harm. Companies like Tesla and Waymo have invested heavily in developing algorithms that aim to make these decisions, but the challenge remains—how do we ensure that these decisions align with societal values?
Let’s not forget about the ethical implications of AI in criminal justice. Predictive policing algorithms are designed to allocate police resources more effectively by predicting where crimes are likely to occur. While the intention is to reduce crime rates and enhance public safety, these systems often rely on historical crime data, which can be biased. This brings us back to the fundamental question: does the end justify the means? If an AI system prioritizes certain neighborhoods based on flawed data, it could reinforce existing inequalities rather than serve the greater good. Thus, while the utilitarian approach seeks to optimize outcomes, it can inadvertently perpetuate harm.
To further illustrate the complexities of utilitarian AI, let’s examine a comparative table that highlights different case studies:
Case Study | Utilitarian Goal | Challenges Faced |
---|---|---|
Healthcare AI | Maximize patient outcomes | Data bias leading to unequal treatment |
Self-Driving Cars | Minimize harm in critical decisions | Ethical dilemmas in life-and-death scenarios |
Predictive Policing | Reduce crime rates | Reinforcement of societal inequalities |
In conclusion, while these case studies showcase the potential for utilitarian AI to create positive outcomes, they also underscore the ethical complexities that arise when decisions are left in the hands of algorithms. As we continue to navigate this evolving landscape, it’s crucial to foster discussions around the moral implications of AI, ensuring that the technology we develop serves humanity's best interests.
- What is utilitarianism in AI? Utilitarianism in AI refers to the ethical framework that prioritizes actions that maximize overall happiness or well-being for the greatest number of people.
- How can bias affect AI decision-making? Bias in AI can lead to unfair or discriminatory outcomes, especially if the training data reflects existing societal inequalities.
- Why is human oversight important in AI? Human oversight ensures that ethical considerations are integrated into AI decision-making, helping to prevent harmful consequences.
- Can AI systems be truly objective? While AI aims to be objective, the data and algorithms used can introduce biases, making complete objectivity challenging.
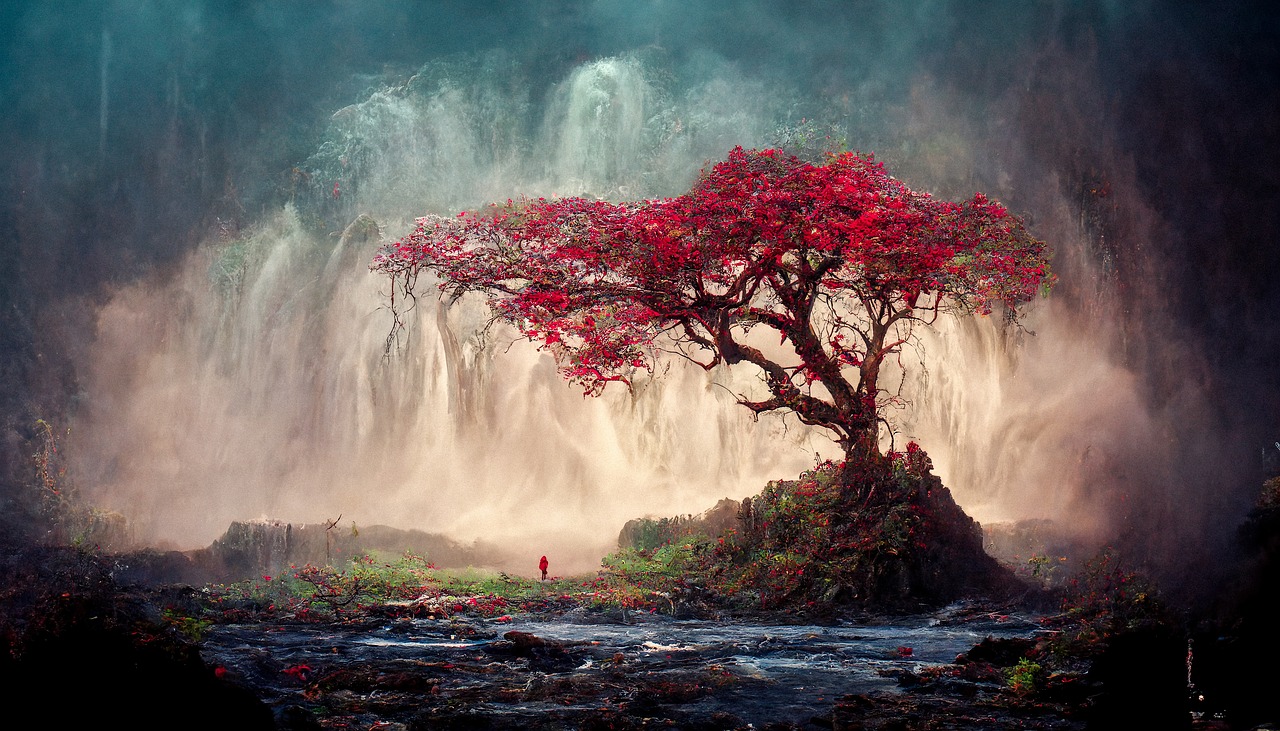
Deontological Ethics and AI
When we dive into the realm of deontological ethics, we encounter a fascinating framework that emphasizes rules and duties over the consequences of actions. In the context of artificial intelligence, this raises some intriguing questions: How can AI systems adhere to moral laws? Can a machine understand the concept of duty? As we explore these questions, we must consider how deontological principles can be integrated into AI decision-making processes.
Deontological ethics, which is often associated with the philosopher Immanuel Kant, posits that actions are morally right if they follow certain rules or duties, regardless of the outcomes they produce. This is a stark contrast to utilitarianism, where the focus is on maximizing overall happiness. In AI, adopting a deontological approach means programming systems to follow predefined ethical guidelines, which can be quite challenging. For instance, imagine an AI designed to make medical decisions. Should it prioritize patient autonomy, even if that decision conflicts with what the AI calculates as the best medical outcome? This is where the complexity of deontological ethics comes into play.
One of the key challenges in implementing deontological ethics in AI is ensuring that these systems can interpret and apply moral rules consistently. Unlike humans, who can navigate complex moral landscapes with emotional intelligence and contextual understanding, AI lacks the innate ability to grasp the nuances of human morality. This limitation can lead to situations where the AI might adhere strictly to a rule but fail to consider the broader implications of its actions. For example, if an AI is programmed to never harm a human, it might refuse to take life-saving actions if they involve any risk, even if the outcome would ultimately save lives.
To better understand how deontological ethics can be applied to AI, we can look at a few essential principles that guide this ethical framework:
- Universalizability: The idea that a moral rule should apply universally. If an AI makes a decision, it should be one that could be accepted as a universal law.
- Respect for Persons: This principle emphasizes treating individuals as ends in themselves, not merely as means to an end. AI systems must be designed to respect human dignity.
- Duty Over Consequence: AI should be programmed to act according to its duties, even when it may lead to less favorable outcomes.
Despite the challenges, the incorporation of deontological ethics into AI is not just a theoretical exercise; it has practical implications. For instance, in the development of autonomous vehicles, programmers face ethical dilemmas about how these vehicles should react in emergency situations. Should an autonomous car prioritize the safety of its passengers over pedestrians? A deontological approach would require the vehicle to adhere to strict rules about human life and safety, potentially leading to more ethical decision-making in critical situations.
Ultimately, the integration of deontological ethics into AI systems could lead to a more structured and principled approach to decision-making. However, it also necessitates ongoing dialogue among ethicists, engineers, and policymakers to ensure that the rules and duties programmed into AI reflect our shared human values. As we advance further into the age of AI, it is imperative that we establish clear guidelines that not only govern the behavior of these systems but also promote accountability and transparency in their operations.
- What is deontological ethics? Deontological ethics is a moral framework that emphasizes the importance of following rules and duties, rather than focusing solely on the consequences of actions.
- How can AI systems apply deontological ethics? AI systems can be programmed with specific moral rules that guide their decision-making processes, ensuring they act in accordance with established ethical principles.
- What are the challenges of implementing deontological ethics in AI? The main challenges include ensuring that AI can interpret and apply moral rules consistently and understanding the complexities of human morality.
- Why is it important for AI to follow ethical guidelines? Following ethical guidelines helps prevent harmful outcomes, promotes fairness, and ensures that AI systems respect human dignity and rights.
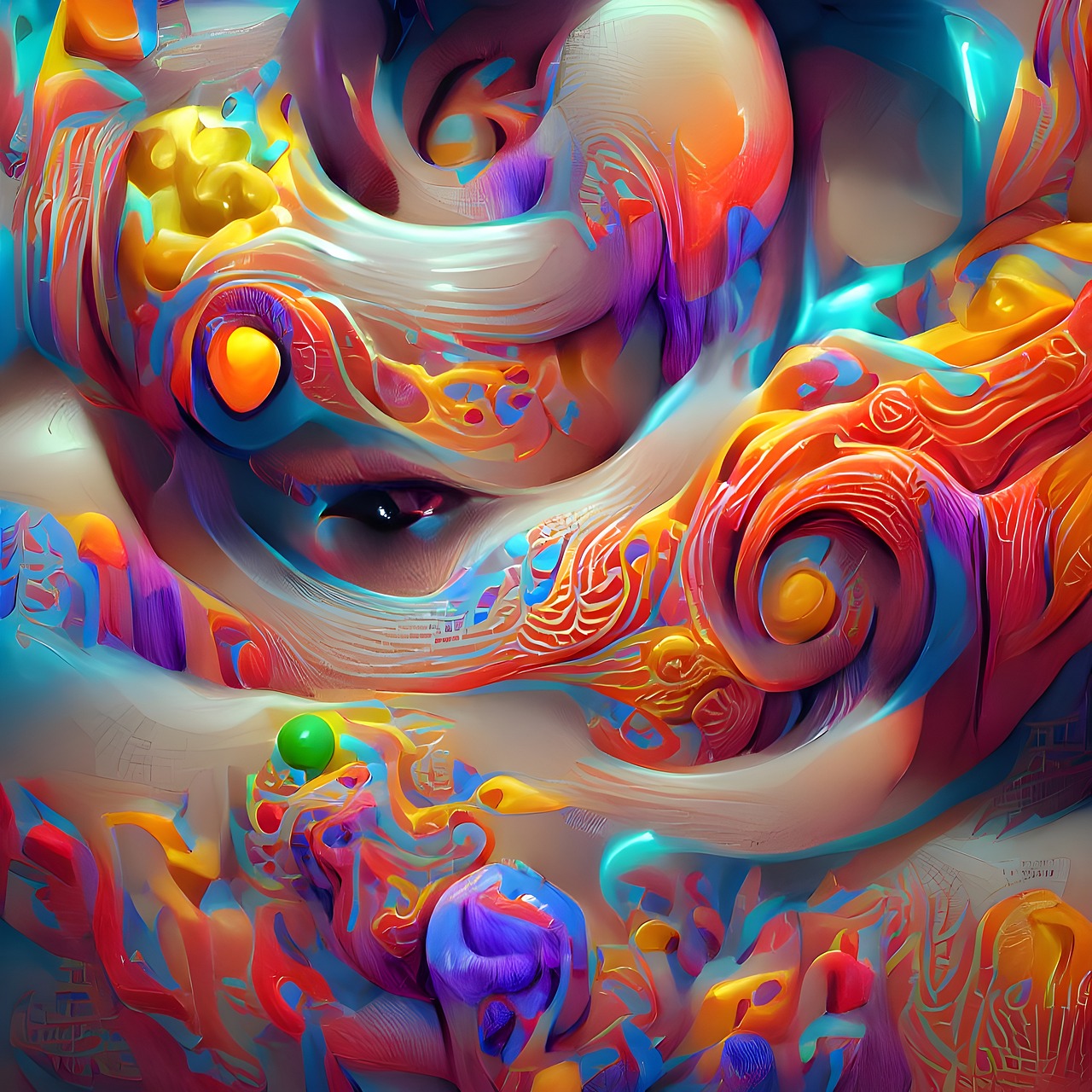
AI Bias and Ethical Implications
Artificial Intelligence (AI) has become an integral part of our daily lives, influencing decisions in various sectors such as healthcare, finance, and law enforcement. However, with its increasing presence comes a significant concern: AI bias. This bias is not merely a technical glitch; it reflects deeper ethical implications that can impact individuals and society at large. When AI systems are trained on historical data, they can inadvertently learn and perpetuate existing prejudices, leading to unfair treatment of certain groups. Imagine a world where a machine decides your fate based on flawed data—it's a chilling thought!
To understand the gravity of AI bias, we must first recognize its sources. Bias can originate from various points in the AI development process, including:
- Data Selection: If the data used to train AI systems is biased, the AI will likely mirror those biases. For instance, if a facial recognition system is primarily trained on images of lighter-skinned individuals, it may perform poorly on people with darker skin tones.
- Algorithm Design: The algorithms themselves can introduce bias. If the criteria for decision-making are not carefully considered, they may favor certain demographics over others.
- Human Oversight: The individuals who design and implement AI systems may unconsciously embed their own biases into the technology.
These biases can lead to serious ethical implications. For example, consider a hiring algorithm that favors candidates from certain universities while disregarding equally qualified applicants from less prestigious institutions. This not only reinforces existing inequalities but also limits diversity in the workplace. The consequences of such biases extend beyond individual cases; they can perpetuate systemic discrimination, exacerbating social divides.
Addressing AI bias is not just about correcting errors; it’s about fostering a more equitable society. To mitigate bias in AI systems, several strategies can be employed:
- Diverse Data Sourcing: Ensuring that training data is representative of the entire population can help create fairer AI systems.
- Algorithmic Transparency: Making AI algorithms transparent allows for scrutiny and understanding of how decisions are made, which can help identify and correct biases.
- Continuous Monitoring: Regularly evaluating AI systems for bias and adjusting them as necessary is crucial for maintaining ethical standards.
In conclusion, the implications of AI bias are profound and far-reaching. As we continue to integrate AI into various aspects of our lives, it is essential to remain vigilant about the ethical considerations that arise. The stakes are high, and the responsibility lies with developers, policymakers, and society as a whole to ensure that AI technology serves to uplift rather than oppress.
- What is AI bias? AI bias refers to the tendency of AI systems to reflect and perpetuate existing prejudices present in their training data.
- How can AI bias impact society? AI bias can lead to unfair treatment of individuals, reinforce societal inequalities, and perpetuate discrimination in various sectors.
- What steps can be taken to mitigate AI bias? Strategies include diverse data sourcing, algorithmic transparency, and continuous monitoring of AI systems.
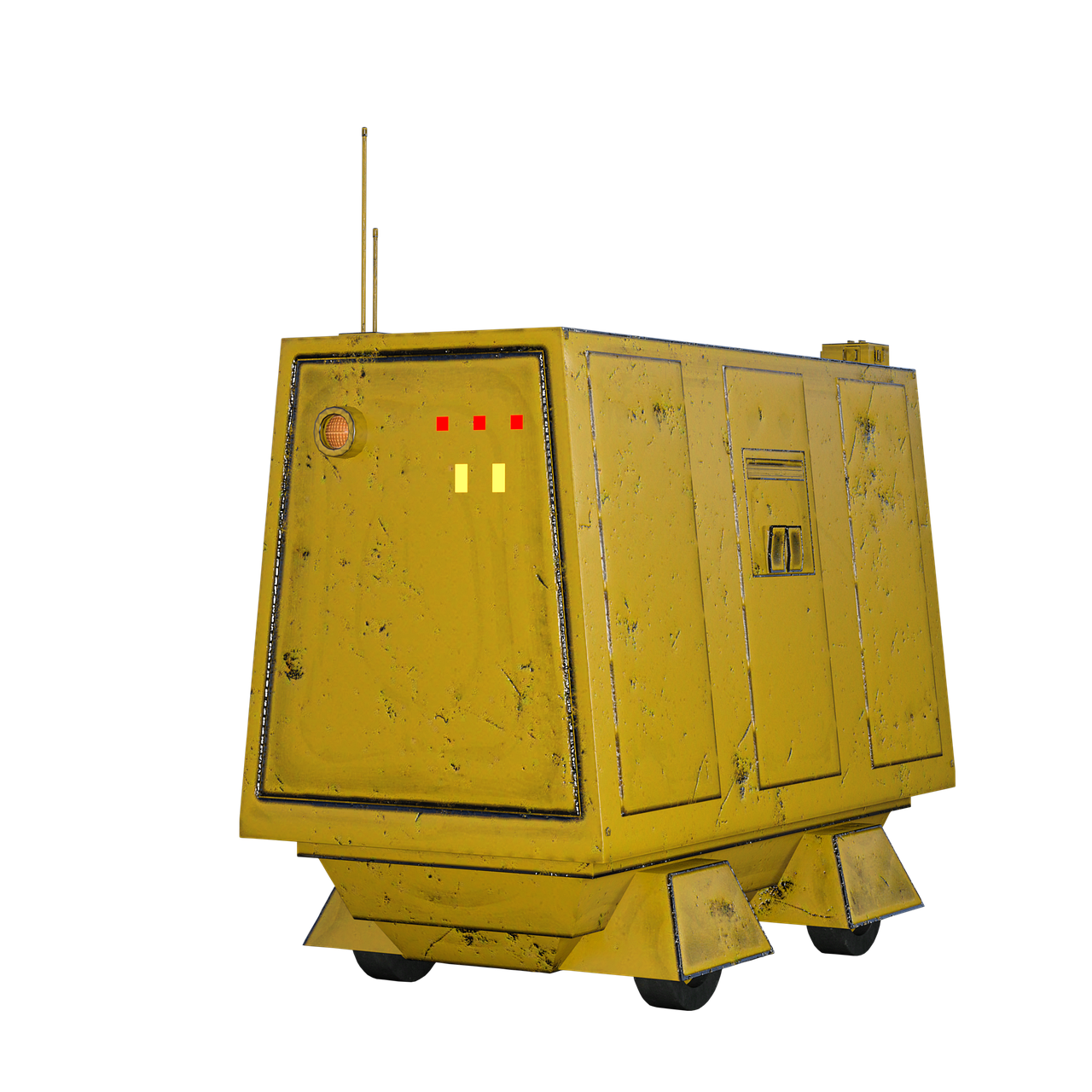
Sources of Bias in AI
When we talk about bias in artificial intelligence, it’s crucial to understand that these biases don't just appear out of thin air. They are often deeply rooted in the data that AI systems are trained on. Think of AI as a sponge; it absorbs everything around it. If the water it soaks up is murky or tainted, the output will be too. This means that the quality of data is paramount in determining how fair and accurate AI systems can be.
One of the primary sources of bias is the selection of training data. If the data used to train an AI model is not representative of the entire population, the AI will inevitably reflect those disparities. For example, if a facial recognition system is primarily trained on images of individuals from one demographic group, its effectiveness will drop significantly when applied to individuals from other groups. This kind of bias can lead to serious ethical implications, especially in areas like law enforcement and hiring practices.
Moreover, the design of algorithms plays a significant role in bias. Algorithms are created by humans, and human biases can inadvertently influence how these algorithms function. For instance, if an algorithm is designed to prioritize certain features based on biased assumptions, it may lead to outcomes that unfairly disadvantage specific groups. This is particularly concerning in sectors like finance, where biased algorithms can lead to discriminatory lending practices.
Another factor contributing to bias is the feedback loop. AI systems often learn from their past decisions and the data they generate. If an AI system is biased in its initial decision-making, it may continue to reinforce those biases over time, creating a cycle that is difficult to break. For example, if a hiring algorithm favors candidates from a particular background, it may lead to fewer opportunities for others, thus perpetuating inequality.
To illustrate the various sources of bias, consider the following table:
Source of Bias | Description |
---|---|
Training Data Selection | Data that is not representative can lead to skewed results. |
Algorithm Design | Human biases in algorithm design can influence outcomes. |
Feedback Loops | Reinforced biases through ongoing learning from flawed data. |
In summary, understanding the sources of bias in AI is essential for creating fair and equitable systems. By scrutinizing training data, algorithm design, and the potential for feedback loops, we can work towards mitigating these biases and fostering a more just technological landscape. The journey towards ethical AI is ongoing, and it requires vigilance and a commitment to continual improvement.
- What is AI bias? AI bias refers to the systematic errors in AI systems that result from prejudiced assumptions in the machine learning process, leading to unfair outcomes.
- How can we mitigate bias in AI? Mitigating bias in AI involves diversifying training data, ensuring transparency in algorithms, and regularly monitoring outcomes to identify and correct biases.
- Why is it important to address bias in AI? Addressing bias in AI is crucial to ensure fairness, accountability, and trust in AI systems, particularly in sensitive applications like healthcare, law enforcement, and hiring.
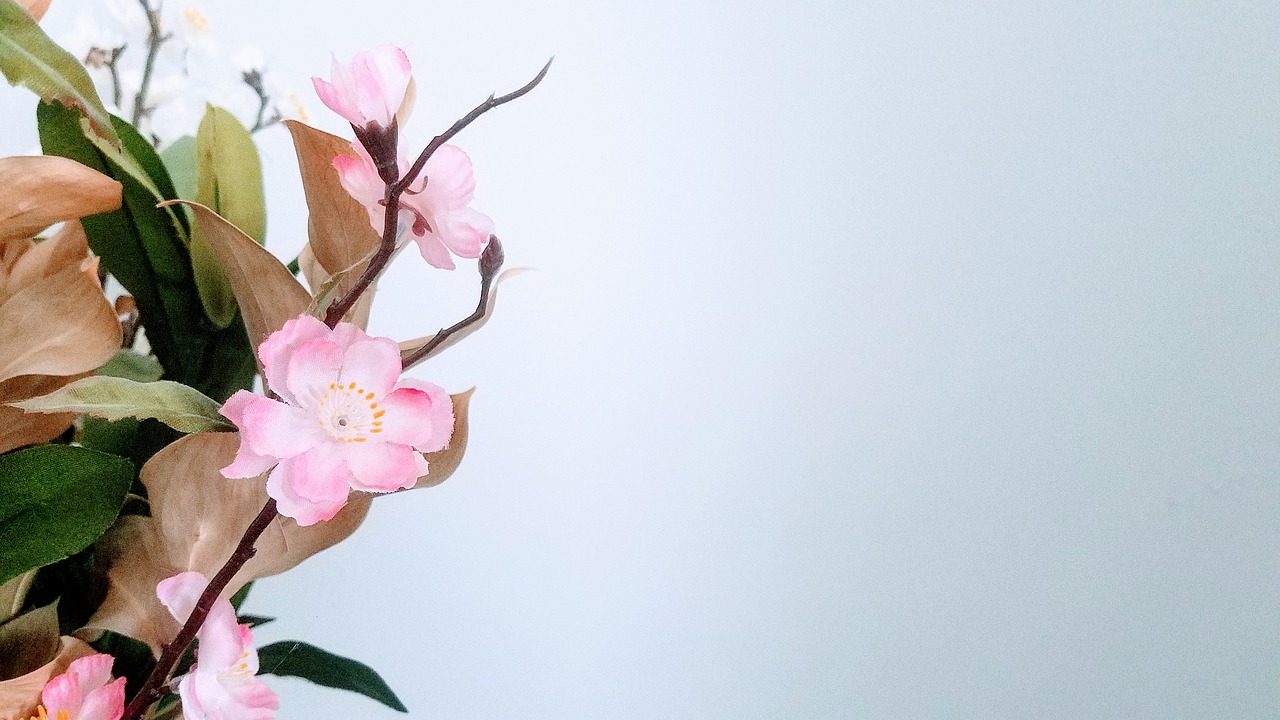
Mitigating Bias in AI Systems
As we navigate the complex landscape of artificial intelligence, one of the most pressing challenges we face is bias. Bias in AI systems can lead to unfair outcomes, perpetuating existing societal inequalities and undermining trust in these technologies. To tackle this issue effectively, it's essential to implement a multi-faceted approach that addresses the sources of bias and fosters fairness. So, how do we go about mitigating bias in AI systems? Let's delve into some strategies that can make a real difference.
One of the first steps in mitigating bias is diverse data sourcing. AI systems learn from the data they are trained on, and if that data is skewed or not representative of the broader population, the AI's decisions will reflect those biases. It's crucial to ensure that the datasets used for training AI models are comprehensive and inclusive. For instance, if an AI system is designed to evaluate job applicants, it should be trained on a diverse set of resumes that represent various backgrounds, experiences, and demographics. This helps to ensure that the AI does not favor one group over another unintentionally.
In addition to diverse data, algorithmic transparency plays a significant role in combating bias. By making the algorithms that power AI systems more transparent, developers and users can better understand how decisions are made. This means that organizations should document their AI development processes, including the data sources, the algorithms used, and the decision-making criteria. Transparency enables stakeholders to scrutinize AI behavior and identify any potential biases that may arise. Accountability is also enhanced when organizations are open about their AI systems.
Continuous monitoring is another vital component in the battle against bias. AI systems should not be viewed as "set it and forget it" solutions. Instead, they require ongoing evaluation to ensure they are functioning fairly. This can involve regularly testing AI outputs against real-world outcomes, seeking feedback from users, and adjusting the algorithms as necessary. For example, if an AI system is found to disproportionately reject applicants from a certain demographic, it should be recalibrated to address this imbalance. By actively monitoring AI systems, organizations can make timely adjustments and foster a culture of ethical AI use.
Finally, it is essential to foster a culture of inclusivity and ethics within organizations that develop AI. This can be achieved by involving diverse teams in the AI development process, encouraging open discussions about ethical implications, and training employees on the importance of fairness in AI. When people from various backgrounds come together, they bring unique perspectives that can help identify and mitigate biases that may not be apparent to a homogenous group.
In conclusion, mitigating bias in AI systems is not just a technical challenge; it's a societal imperative. By prioritizing diverse data sourcing, promoting algorithmic transparency, engaging in continuous monitoring, and fostering an inclusive culture, we can work towards developing AI systems that are fair, accountable, and reflective of our diverse world. The journey may be complex, but the rewards—greater trust in AI and a more equitable society—are undoubtedly worth the effort.
- What is AI bias? AI bias refers to systematic and unfair discrimination in AI systems, often stemming from biased training data or flawed algorithms.
- How can bias in AI be identified? Bias can be identified through testing AI outputs against diverse datasets and conducting audits on AI decision-making processes.
- What role does human oversight play in AI? Human oversight is crucial to ensure ethical considerations are integrated into AI decision-making and to hold systems accountable for their actions.
- Can AI be completely unbiased? While it may be challenging to achieve complete bias-free AI, implementing strategies for mitigation can significantly reduce the impact of bias.
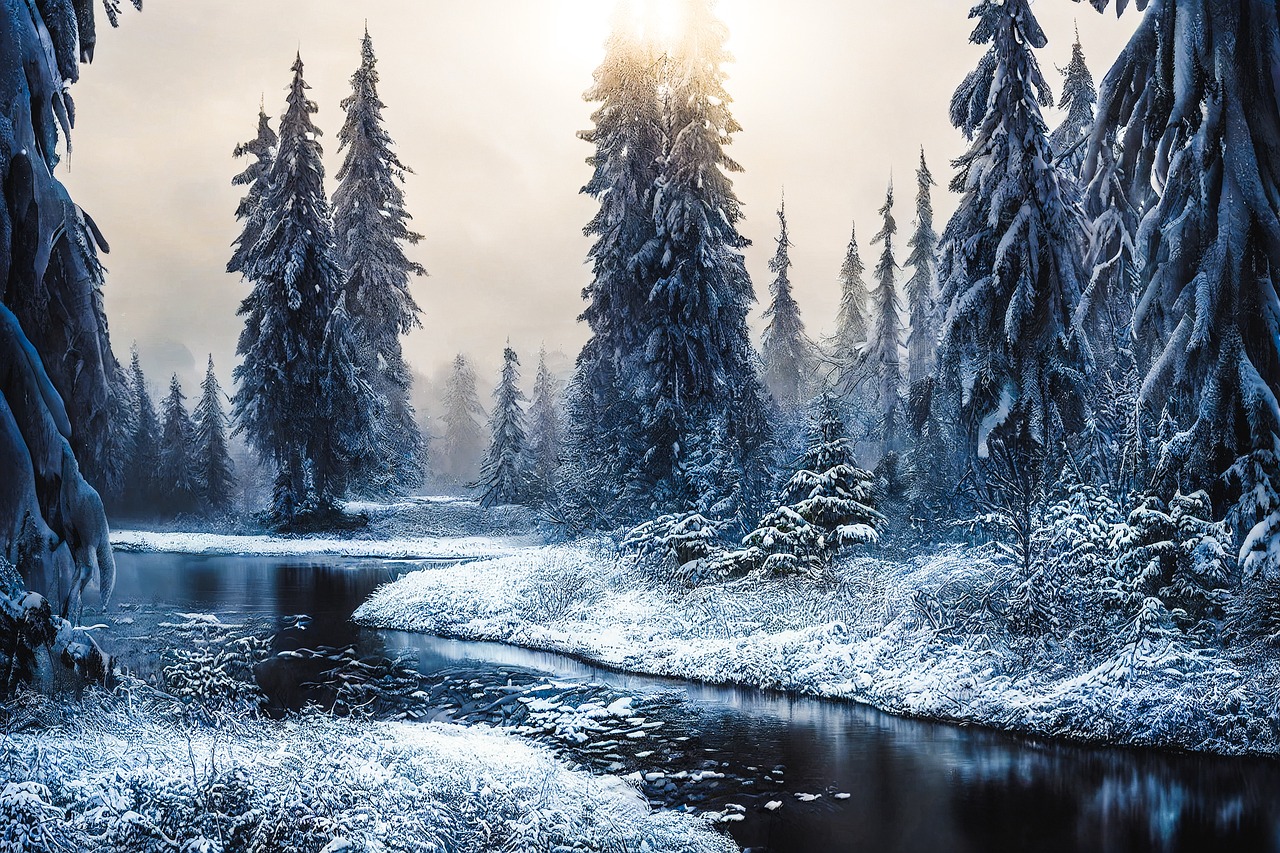
The Role of Human Oversight
In the rapidly evolving landscape of artificial intelligence (AI), the importance of human oversight cannot be overstated. As AI systems become more sophisticated and autonomous, the question arises: who is responsible for the decisions made by these systems? It's vital to understand that while AI can process data and make decisions at lightning speed, it lacks the nuanced understanding of human values, ethics, and emotional intelligence. This is where human oversight comes into play, acting as a crucial checkpoint in the AI decision-making process.
Imagine you’re driving a car equipped with advanced autopilot features. While the technology can navigate traffic and adjust speeds, it still requires a human driver to monitor the road and make judgment calls in unpredictable situations. Similarly, AI systems need a human touch to ensure that ethical considerations are woven into their fabric. Without this oversight, we risk allowing machines to make decisions that could lead to harmful or unintended consequences.
Moreover, human oversight fosters accountability in AI systems. When AI makes a decision, it’s essential to have mechanisms in place that clarify who is responsible for those choices. This accountability can be established through clear governance frameworks and ethical guidelines that dictate how AI should operate in various contexts. For instance, in sectors like healthcare or criminal justice, the implications of AI decisions can be profound, affecting lives and communities. Therefore, having humans involved in the oversight process ensures that there is a layer of responsibility that machines simply cannot provide.
Furthermore, collaboration between humans and AI can enhance decision-making processes. Rather than viewing AI as a replacement for human judgment, we should see it as a complementary tool. For example, in fields like finance, AI can analyze vast amounts of data to identify trends and risks, but it is the financial analyst who interprets these insights and makes strategic decisions. This partnership can lead to better outcomes, as both AI's computational power and human intuition are leveraged effectively.
To illustrate the significance of human oversight, consider the following table that outlines the benefits of integrating human oversight into AI systems:
Benefits of Human Oversight | Description |
---|---|
Ethical Decision-Making | Ensures that AI decisions align with human values and ethical standards. |
Accountability | Establishes clear lines of responsibility for AI-driven decisions. |
Enhanced Collaboration | Combines AI's analytical capabilities with human intuition for better outcomes. |
Risk Mitigation | Helps identify and address potential biases and errors in AI systems. |
In conclusion, while AI has the potential to revolutionize various industries, the need for human oversight is paramount. As we continue to integrate AI into our daily lives, we must prioritize ethical considerations and ensure that human values remain at the forefront of technological advancements. This collaborative approach not only enhances the effectiveness of AI systems but also safeguards against the risks associated with autonomous decision-making.
- Why is human oversight necessary in AI? Human oversight is crucial to ensure that AI decisions align with ethical standards and human values, preventing potential harm.
- How can we establish accountability for AI decisions? Clear governance frameworks and ethical guidelines can help clarify responsibilities for decisions made by AI systems.
- Can AI and humans work together effectively? Yes, AI can complement human judgment by providing data-driven insights, leading to better decision-making outcomes.
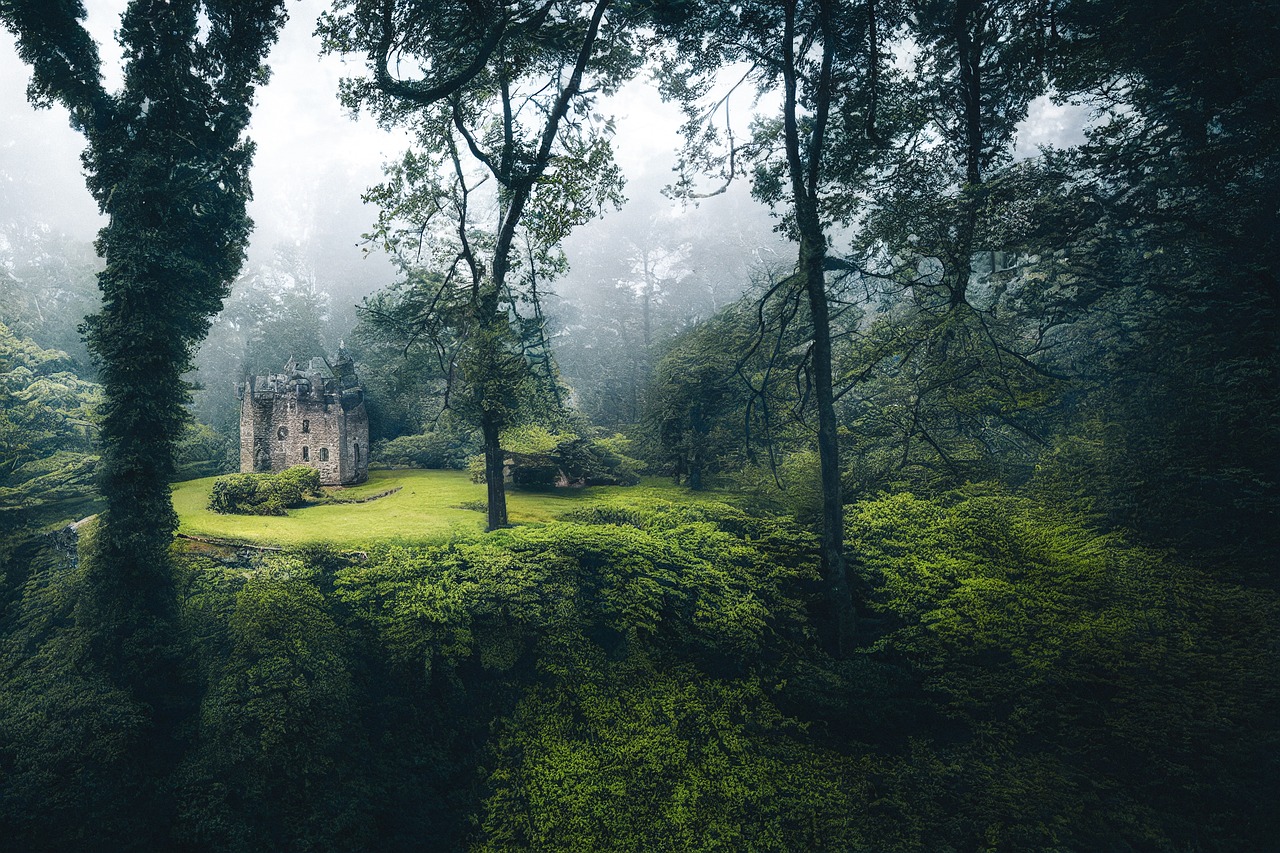
Accountability in AI Decisions
In the rapidly evolving landscape of artificial intelligence, the concept of accountability is becoming increasingly crucial. As AI systems take on more significant roles in decision-making processes, the question arises: who is responsible when things go wrong? This dilemma is not just a theoretical exercise; it has real-world implications that can affect individuals, organizations, and even entire societies. Imagine a scenario where an autonomous vehicle makes a decision that leads to an accident. Who bears the responsibility? The manufacturer, the software developer, or the AI itself? These questions highlight the urgent need for clear accountability frameworks in AI.
One of the primary challenges in establishing accountability in AI decisions lies in the **complexity** of these systems. AI operates on algorithms that can be intricate and opaque, making it difficult to trace the reasoning behind a specific decision. This lack of transparency can lead to a phenomenon known as the "black box" problem, where the inner workings of AI remain hidden even from those who designed them. To tackle this issue, experts advocate for enhanced algorithmic transparency, which can help stakeholders understand how decisions are made. By shedding light on the decision-making process, we can better assign responsibility and ensure that ethical considerations are at the forefront.
Moreover, the concept of accountability in AI also necessitates a robust regulatory framework. Governments and regulatory bodies must step in to create guidelines that define the responsibilities of AI developers and users. This could involve establishing standards for ethical AI use, ensuring that companies are held accountable for the outcomes of their AI systems. A potential model for this could be the **General Data Protection Regulation (GDPR)** in Europe, which emphasizes data protection and privacy. By drawing parallels between data accountability and AI accountability, we can begin to forge a path toward responsible AI deployment.
Additionally, it’s essential to recognize that accountability in AI is not solely a matter of legal frameworks; it also involves a cultural shift within organizations. Companies should foster a culture of accountability where ethical considerations are integrated into the AI development lifecycle. This means involving diverse teams in the design and implementation of AI systems, ensuring that various perspectives are considered. A diverse team can better identify potential biases and ethical dilemmas, leading to more responsible AI outcomes.
Ultimately, accountability in AI decisions is about more than just assigning blame; it’s about creating a system where ethical standards are upheld, and human values are prioritized. As we navigate this complex terrain, we must remain vigilant and proactive in addressing the ethical implications of AI. The future of AI is not just about technological advancement; it’s also about ensuring that these advancements serve humanity in a fair and just manner. By establishing clear accountability mechanisms, we can pave the way for a future where AI enhances our lives without compromising our ethical standards.
- What is accountability in AI?
Accountability in AI refers to the responsibility of individuals or organizations for the decisions made by AI systems, ensuring that ethical standards are upheld. - Why is accountability important in AI?
Accountability is crucial to prevent harm, ensure transparency, and maintain public trust in AI technologies. - How can we improve accountability in AI?
By enhancing algorithmic transparency, establishing regulatory frameworks, and fostering a culture of ethical responsibility within organizations. - What is the "black box" problem?
The "black box" problem refers to the difficulty in understanding how AI systems make decisions, often due to the complexity and opaqueness of their algorithms.
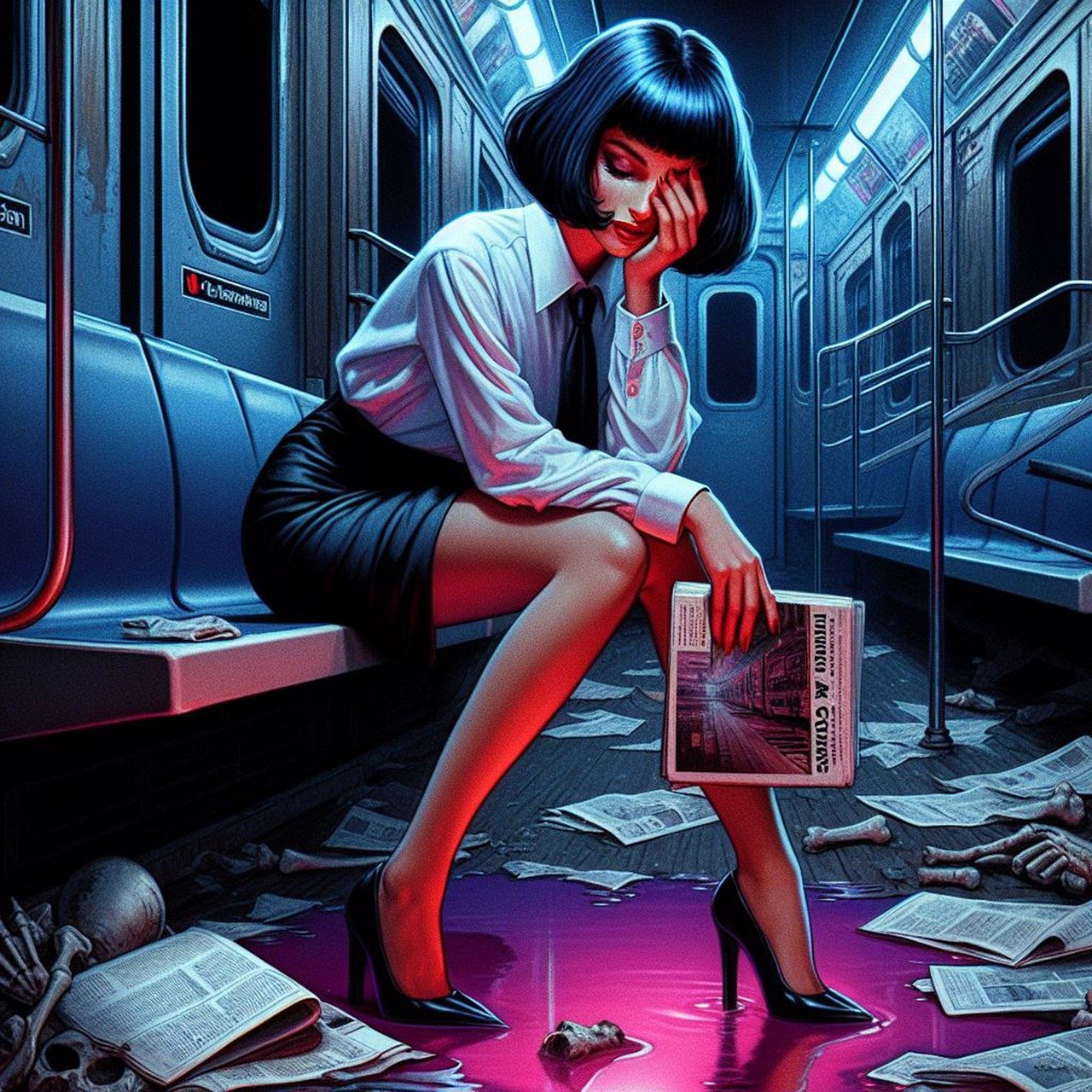
Collaboration Between AI and Humans
In the rapidly evolving landscape of technology, the collaboration between artificial intelligence (AI) and humans is not just a possibility; it’s becoming a necessity. Imagine a world where machines don’t just operate in isolation but work alongside humans, enhancing our capabilities and making our lives easier. This partnership can lead to remarkable outcomes, but it also raises important questions about how we can effectively integrate AI into our daily decision-making processes.
One of the most compelling aspects of this collaboration is the potential for AI to handle data-driven tasks that are often too complex for humans to manage alone. For instance, in the healthcare sector, AI algorithms can analyze vast amounts of medical data to identify patterns that may elude even the most experienced doctors. By doing so, they can assist in diagnosing diseases earlier and more accurately. However, it’s crucial to remember that while AI can process information at lightning speed, it lacks the human touch—something that is invaluable in fields like healthcare, where empathy and ethical considerations are paramount.
The synergy between AI and humans can also be seen in creative industries. AI tools are now being used to generate music, art, and even literature. For example, AI algorithms can analyze existing works to create new compositions or suggest enhancements to an artist's creation. This raises an intriguing question: can AI truly be considered a collaborator, or is it merely a tool? The answer likely lies in how we choose to utilize these technologies. By viewing AI as a partner rather than a replacement, we can foster an environment where creativity flourishes.
However, the collaboration doesn’t come without its challenges. There is a growing concern about the potential for AI to overshadow human judgment. If we rely too heavily on AI systems, we may inadvertently cede our decision-making power. This is where the importance of human oversight comes into play. It’s essential for humans to remain actively involved in the decision-making process, ensuring that ethical considerations are prioritized and that the nuances of human experience are not overlooked.
To facilitate a productive collaboration, organizations need to focus on a few key areas:
- Training and Education: Providing training for employees on how to effectively work with AI tools can lead to better outcomes and a more harmonious partnership.
- Transparency: Ensuring that AI systems are transparent in their processes helps build trust and allows humans to understand the rationale behind AI-driven decisions.
- Feedback Loops: Establishing mechanisms for feedback can help refine AI systems and improve their alignment with human values and expectations.
In conclusion, the collaboration between AI and humans holds incredible promise for the future. By embracing this partnership and addressing the challenges that come with it, we can harness the strengths of both humans and machines. This not only enhances our capabilities but also ensures that our ethical standards are upheld in an increasingly automated world. The journey toward a harmonious collaboration is just beginning, and it is up to us to navigate it wisely.
Q1: How can AI improve collaboration with humans?
A1: AI can enhance collaboration by processing large amounts of data quickly, providing insights, and automating routine tasks, allowing humans to focus on more complex and creative aspects of their work.
Q2: What are the ethical implications of AI collaboration?
A2: The ethical implications include concerns about accountability, transparency, and the potential for AI to make decisions that may overlook human values and nuances, necessitating human oversight.
Q3: Can AI replace human creativity?
A3: While AI can assist in creative processes, it lacks the emotional depth and unique perspective that humans bring. Therefore, AI is better viewed as a tool that complements human creativity rather than a replacement.
Frequently Asked Questions
- Can artificial intelligence truly have a moral compass?
While AI can be programmed to follow certain ethical guidelines, it lacks the innate understanding of morality that humans possess. AI operates based on algorithms and data, which means it doesn't have feelings or consciousness to guide its decisions like a moral compass would.
- What ethical frameworks are used in AI development?
There are several ethical frameworks that guide AI development, including utilitarianism, which focuses on the greatest good for the greatest number, and deontological ethics, which emphasizes adherence to rules and duties. These frameworks influence how AI systems prioritize decisions and human values.
- How does bias affect AI decision-making?
Bias in AI can stem from various sources, such as biased training data or flawed algorithms. This can lead to unfair outcomes, discrimination, and the reinforcement of societal inequalities, raising significant ethical concerns about the fairness of AI systems.
- What are the challenges of implementing utilitarian principles in AI?
Implementing utilitarianism in AI is complex because it requires accurate predictions of outcomes and the ability to measure happiness or suffering. This can be difficult, as the consequences of AI decisions are often uncertain and multifaceted.
- How can we mitigate bias in AI systems?
To reduce bias in AI, strategies such as sourcing diverse data, ensuring algorithmic transparency, and conducting continuous monitoring are essential. These approaches help create fairer and more equitable AI systems that align better with ethical standards.
- Why is human oversight important in AI?
Human oversight is crucial in AI decision-making to ensure that ethical considerations are integrated into the process. This oversight helps prevent harmful outcomes and maintains accountability, ensuring that AI systems align with human values.
- What role does collaboration between AI and humans play?
Collaboration between AI and humans can enhance decision-making by allowing AI to complement human judgment rather than replace it. This synergy can lead to more ethical and effective outcomes across various fields, ensuring that human values remain central.