How the Philosophy of Chance Defines Probability in Science
The intricate dance between chance and probability is not just a mathematical game; it’s a profound philosophical inquiry that shapes our understanding of the universe. When we think about probability, we often picture dice rolling or cards being shuffled; however, the implications of chance extend far beyond mere games of luck. This article delves into how philosophical perspectives on chance influence scientific methodologies and interpretations of probability, weaving a narrative that connects ancient wisdom with modern scientific inquiry.
At its core, the philosophy of chance raises fundamental questions: Is the universe inherently deterministic, or is randomness a vital component of existence? These questions have puzzled thinkers from Aristotle to contemporary scientists. Understanding this relationship is crucial because it informs how we interpret data, make predictions, and ultimately understand the world around us.
As we explore the philosophical underpinnings of probability, we uncover layers of complexity that reveal the significance of randomness in scientific inquiry. For instance, in fields like quantum mechanics, probability is not just a tool; it’s a foundational element that drives our understanding of atomic behavior. Here, chance isn't merely an afterthought; it’s a central player in the drama of existence, where particles behave unpredictably, and outcomes are often probabilistic rather than deterministic.
Moreover, the philosophical debate surrounding determinism versus indeterminism plays a pivotal role in shaping our understanding of probability. Determinists argue that every event is predetermined by preceding events, leaving little room for chance. On the other hand, indeterminists embrace the idea that randomness is an intrinsic part of the universe, leading to a more nuanced interpretation of probability. This dichotomy influences various scientific disciplines, from physics to biology, and challenges researchers to reconsider how they approach their studies.
In addition to the philosophical debates, we also encounter the distinction between subjective and objective probability. Subjective probability hinges on personal belief and interpretation, while objective probability is grounded in empirical evidence and statistical analysis. This distinction is crucial for scientists as they navigate the complexities of research, making decisions based on either their intuition or hard data. Understanding these perspectives can significantly impact the outcomes of scientific studies and the conclusions drawn from them.
As we venture into the practical applications of probability in science, we find that its relevance spans a wide array of fields. From predicting weather patterns to modeling the spread of diseases, probability is a critical tool that scientists employ to make sense of uncertainty. For example, in epidemiology, probability models are essential for understanding the likelihood of disease outbreaks and the effectiveness of interventions. These applications not only highlight the importance of probability but also underscore how philosophical interpretations of chance can influence real-world outcomes.
However, despite its significance, comprehending and applying probability is fraught with challenges. Misconceptions about randomness often lead to flawed interpretations and misguided conclusions. For instance, many people fall prey to the gambler's fallacy, believing that past events influence future outcomes in random processes. Addressing these misconceptions is vital for enhancing the accuracy of scientific interpretations and applications.
Furthermore, the complexity of probabilistic models can pose significant challenges for scientists. These models often require a deep understanding of both mathematical principles and the philosophical implications of chance. As scientific inquiry continues to evolve, the interplay between philosophical perspectives and probabilistic models will undoubtedly shape future discoveries and methodologies.
In conclusion, the philosophy of chance is not just an abstract concept; it’s a fundamental aspect of how we interpret probability in science. By understanding the philosophical dimensions of chance, we can better appreciate the role of probability in scientific inquiry and its implications for our understanding of the universe. As we continue to explore this fascinating relationship, we must remain open to the surprises and complexities that chance presents, embracing the uncertainty that is inherent in the scientific process.
- What is the difference between chance and probability? Chance refers to the likelihood of an event occurring, while probability quantifies that likelihood mathematically.
- How does randomness affect scientific experiments? Randomness introduces variability in experiments, making it essential for scientists to account for it when interpreting results.
- Can probability predict future events accurately? Probability provides a framework for estimating outcomes, but it cannot guarantee specific results due to inherent uncertainties.
- Why is the philosophy of chance important in science? It influences how scientists interpret data and make predictions, shaping the methodologies used in various research fields.
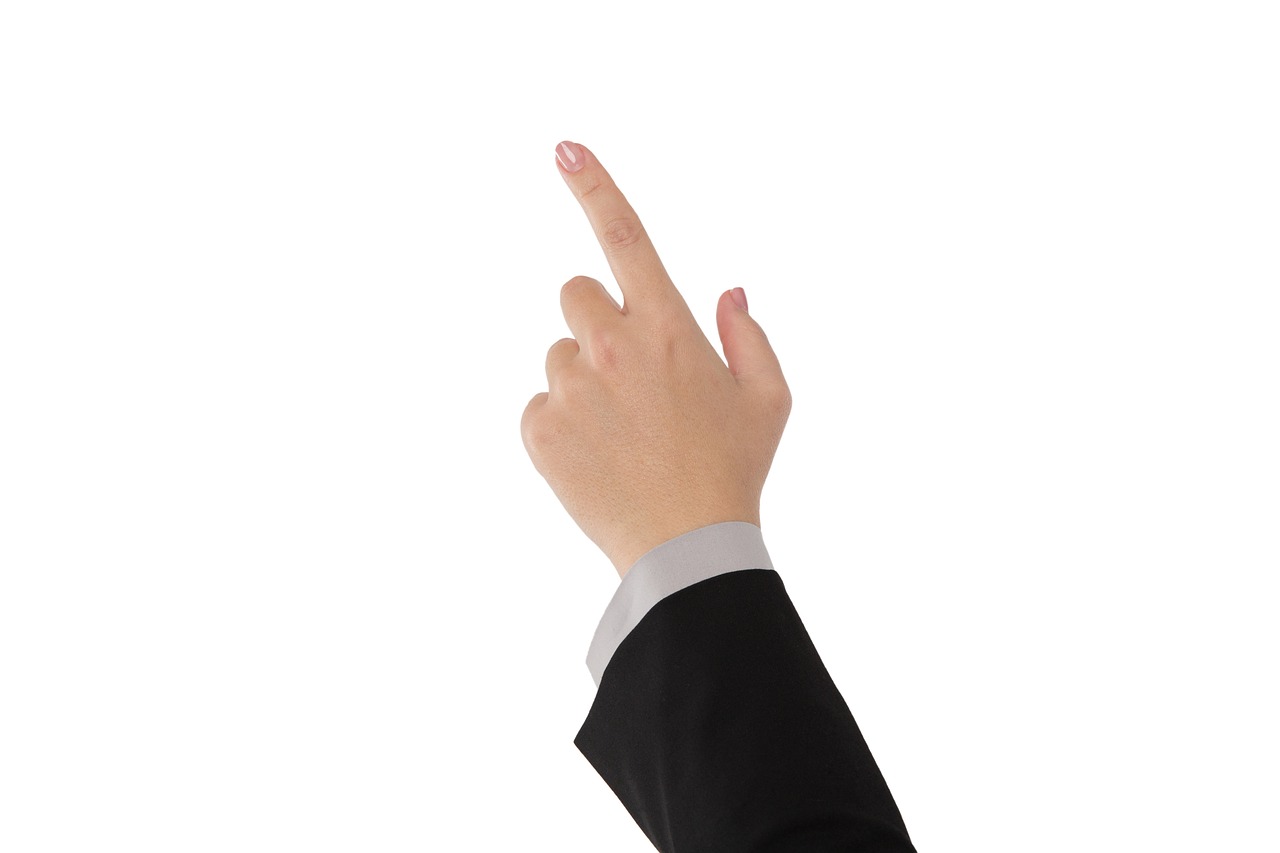
The Historical Context of Chance
Understanding the evolution of the concept of chance provides profound insight into its significance in probability theory and scientific inquiry. The journey of chance begins in ancient philosophies, where thinkers like Aristotle grappled with the unpredictability of events. He believed that while some occurrences were governed by natural laws, others fell into the realm of chance, a notion that laid the groundwork for future explorations into randomness.
As we move through history, the Renaissance period marked a pivotal shift. Scholars began to embrace a more mathematical approach to chance, leading to the birth of probability theory. The works of mathematicians such as Gerolamo Cardano and Blaise Pascal introduced formal methods for calculating probabilities, transforming chance from a philosophical concept into a quantitative discipline. Cardano's writings on gambling strategies and Pascal's correspondence with Pierre de Fermat on problems of dice games were instrumental in establishing the foundational principles of probability.
In the 17th century, the concept of randomness began to take shape. The idea that events could be inherently random sparked debates among philosophers and scientists alike. Some argued that randomness was merely a reflection of our ignorance, while others posited that true randomness existed in nature. This philosophical divide set the stage for modern interpretations of probability, influencing how scientists approached the analysis of uncertain events.
Fast forward to the 20th century, where the development of statistical mechanics and quantum theory further complicated our understanding of chance. Figures like Albert Einstein and Niels Bohr engaged in heated debates over the implications of randomness in quantum mechanics. Einstein famously declared, “God does not play dice with the universe,” reflecting his discomfort with the indeterministic nature of quantum events. This clash of ideas highlighted the ongoing tension between deterministic and probabilistic interpretations of the universe.
Today, the historical context of chance continues to evolve, shaping our understanding of probability across various fields. The philosophical perspectives on chance influence not only scientific theories but also practical applications in areas such as finance, healthcare, and artificial intelligence. As we explore the intricate relationship between chance and probability, it becomes evident that our historical journey is not just a tale of mathematical advancement but a profound inquiry into the nature of reality itself.
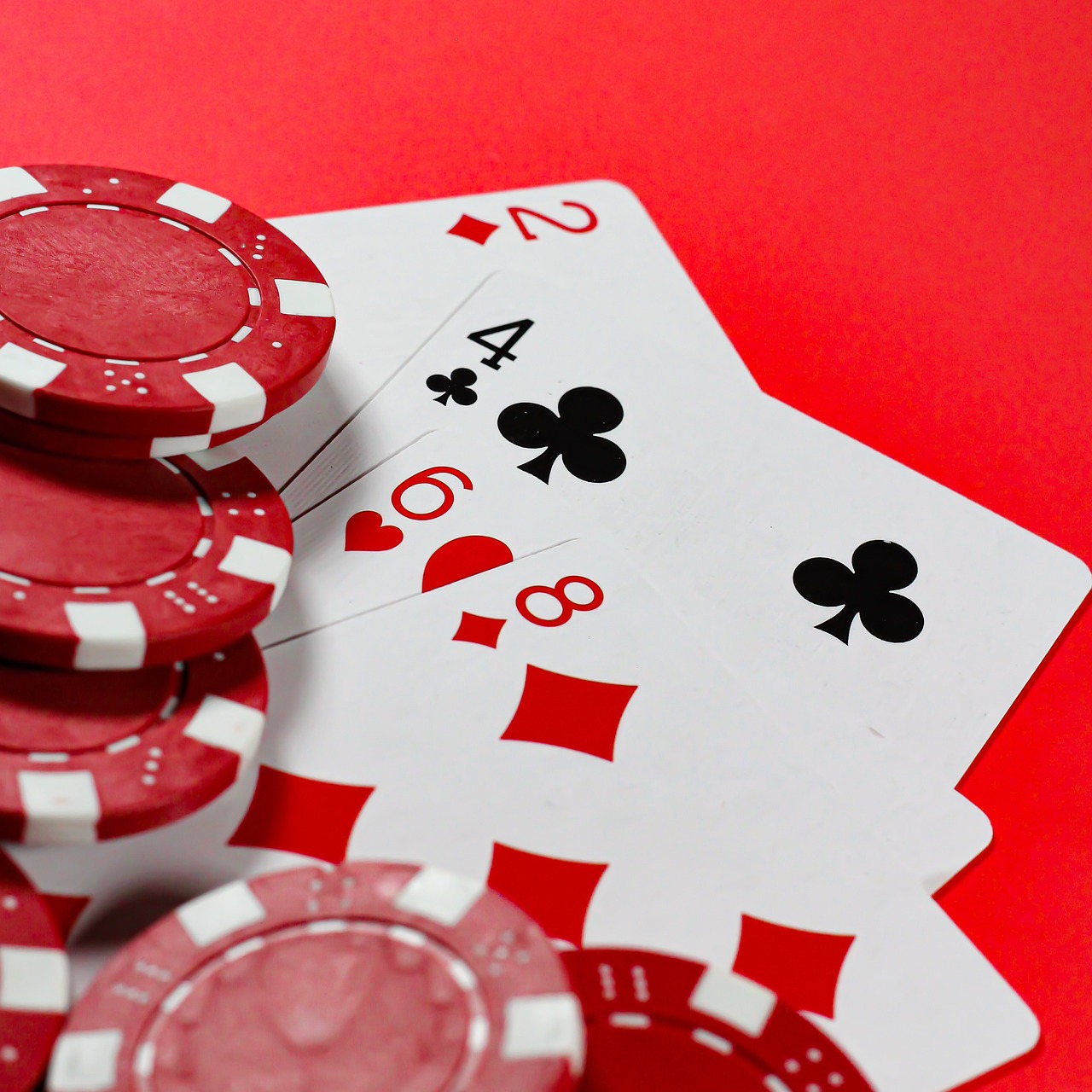
The Role of Randomness in Probability
Randomness is often viewed as the chaotic dance of chance, an unpredictable force that shapes outcomes in both everyday life and scientific inquiry. In the realm of probability, randomness is not merely an afterthought; it is a fundamental pillar that influences how we interpret data and make predictions. Think of randomness as the wild card in a game of poker – it can change the entire outcome based on factors we cannot control. This unpredictability is essential in various scientific experiments, where it serves to highlight the inherent uncertainties in measurements and observations.
To grasp the significance of randomness in probability, we first need to define what randomness actually is. At its core, randomness refers to the lack of pattern or predictability in events. In probability theory, it is often quantified and measured, allowing scientists to draw conclusions based on observed frequencies and distributions. For instance, when flipping a coin, the outcome is random; however, over a large number of flips, the probability of landing on heads or tails converges to 50%. This convergence illustrates how randomness can be tamed through statistical analysis, revealing underlying patterns that might initially seem chaotic.
Moreover, randomness plays a crucial role in various scientific contexts. Here are a few areas where randomness is pivotal:
- Quantum Mechanics: In quantum physics, particles exhibit behavior that is intrinsically random. The position and momentum of particles can only be predicted probabilistically, leading to the famous Heisenberg Uncertainty Principle.
- Epidemiology: Randomness is vital in understanding the spread of diseases. Random sampling methods help researchers gather data that can lead to insights about transmission patterns and risk factors.
- Weather Forecasting: Meteorologists use probabilistic models to predict weather patterns, acknowledging the inherent randomness in atmospheric conditions that can lead to sudden changes.
Despite its chaotic nature, randomness is not entirely devoid of structure. Scientists have developed various methods to analyze and interpret random phenomena, such as statistical distributions and probabilistic models. These tools allow researchers to make informed predictions based on random events, transforming chaos into clarity. For example, the normal distribution curve, often depicted as a bell curve, illustrates how random variables tend to cluster around a mean, demonstrating that while individual outcomes may be random, collective behaviors can be predicted.
However, the measurement of randomness is not without its challenges. The complexity of probabilistic models can sometimes obscure the true nature of randomness, leading to misconceptions about how probability operates in real-world scenarios. For instance, many people fall into the gambler's fallacy, believing that past outcomes can influence future events in independent trials. Understanding that each coin flip is independent of the previous ones is essential for accurately interpreting randomness.
In conclusion, randomness is a double-edged sword in the field of probability. It introduces uncertainty and unpredictability, yet it also provides the foundation for statistical reasoning and scientific inquiry. By embracing randomness, scientists can uncover patterns and make predictions that enhance our understanding of the world around us. As we continue to explore the intricate relationship between chance and probability, the role of randomness will undoubtedly remain a key area of focus in both philosophical and scientific discussions.
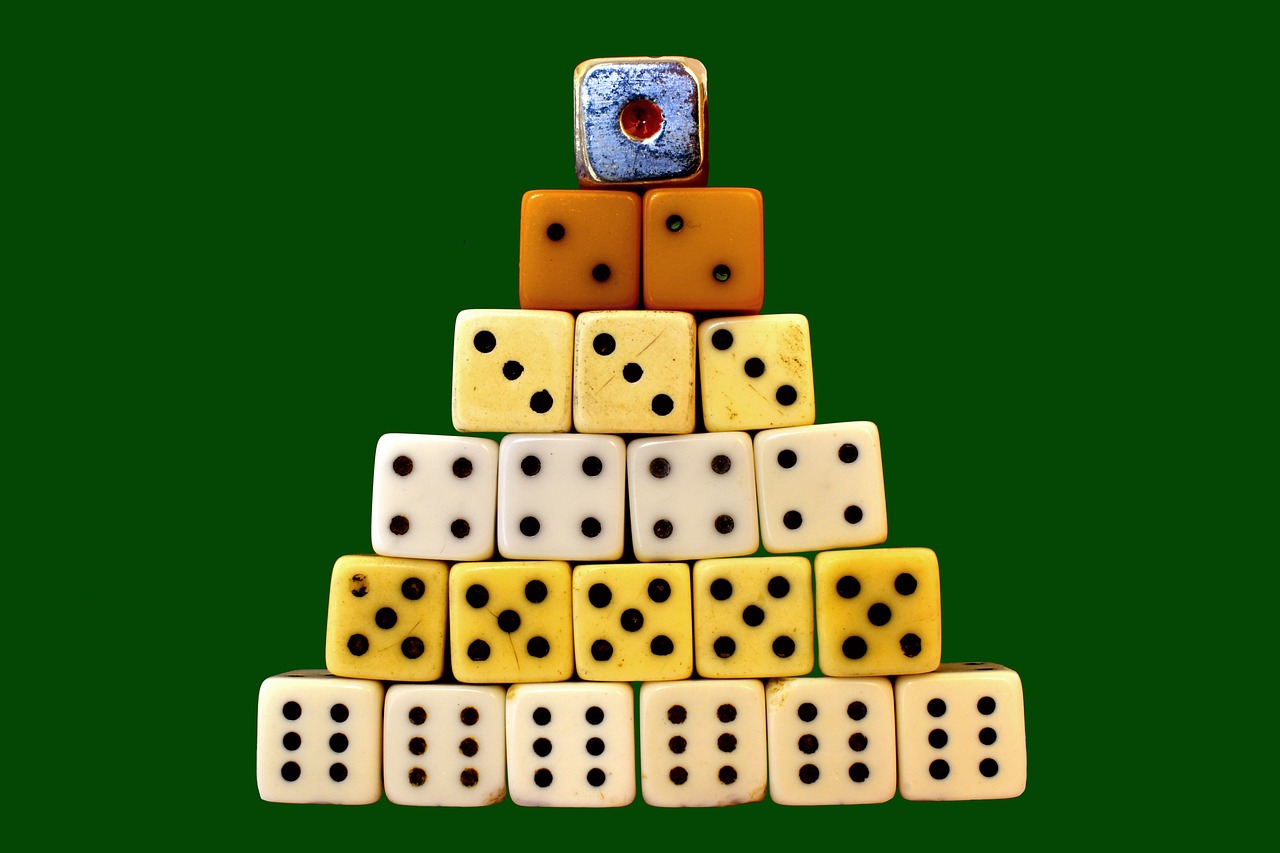
Philosophical Perspectives on Randomness
The concept of randomness has intrigued philosophers for centuries, sparking debates that delve deep into the nature of reality itself. At its core, randomness challenges our understanding of causality and predictability, leading to various interpretations that influence scientific thought. Imagine standing at the edge of a vast ocean, where each wave represents a different philosophical viewpoint—some crashing with certainty, while others drift unpredictably. This analogy captures the essence of how randomness is perceived across various schools of thought.
One prominent perspective is the **deterministic view**, which posits that every event or outcome is the result of preceding causes, leaving little room for randomness. Think of it as a well-oiled machine, where every cog and gear operates in a predictable manner. In this framework, randomness is merely a reflection of our ignorance; if we had complete knowledge of all variables, we could predict any outcome with absolute certainty. This viewpoint aligns closely with classical physics, where the universe is seen as a clockwork mechanism, governed by natural laws.
On the flip side, we have the **indeterministic perspective**, which embraces randomness as a fundamental aspect of the universe. This view suggests that some events occur without any deterministic cause, akin to rolling a die—each roll is independent, and the outcome is inherently uncertain. Quantum mechanics exemplifies this perspective, where particles exist in a state of probability rather than certainty. The famous thought experiment, Schrödinger's cat, illustrates this beautifully: the cat is simultaneously alive and dead until observed, highlighting the role of chance in the very fabric of reality.
Additionally, the distinction between **subjective and objective probability** further complicates our understanding of randomness. Subjective probability is based on personal belief and experience, making it inherently variable. For instance, two people might assess the likelihood of rain tomorrow differently based on their past experiences or feelings about the weather. In contrast, objective probability relies on empirical data and mathematical models, offering a more standardized approach. This divergence raises questions about how we interpret randomness in scientific research and everyday decision-making.
Ultimately, the philosophical perspectives on randomness serve as a lens through which we can examine the complexities of probability in science. By acknowledging these diverse viewpoints, we can appreciate the rich tapestry of ideas that shape our understanding of chance. Whether we lean towards determinism or embrace the chaos of indeterminism, the dialogue surrounding randomness continues to evolve, challenging us to rethink our assumptions about the universe.
- What is randomness? Randomness refers to the lack of pattern or predictability in events. In probability, it signifies outcomes that cannot be precisely determined.
- How does randomness affect scientific experiments? Randomness introduces variability in experiments, which can influence results and interpretations. Understanding this variability is crucial for accurate data analysis.
- What is the difference between subjective and objective probability? Subjective probability is based on personal judgment, while objective probability is derived from empirical evidence and statistical analysis.
- Can randomness be measured? Yes, randomness can be quantified through statistical methods, allowing scientists to assess the likelihood of various outcomes.
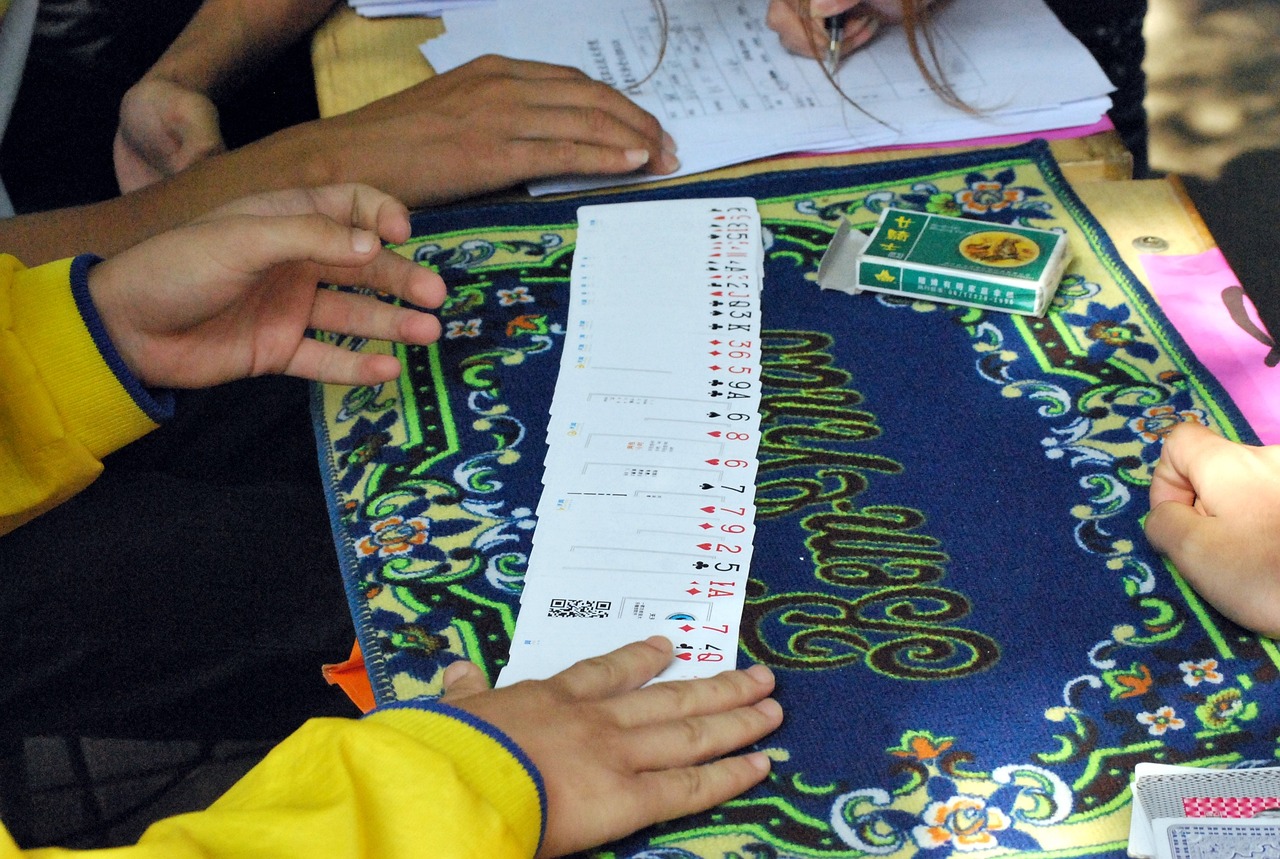
Determinism vs. Indeterminism
When we dive into the philosophical waters of determinism and indeterminism, we find ourselves navigating through a sea of ideas that challenge our understanding of chance and probability. Determinism posits that every event or action is determined by preceding events in accordance with the laws of nature. In this view, the universe operates like a giant clockwork mechanism, where each tick is predetermined and every outcome is merely the result of prior states. Imagine a game of chess: if you know the position of every piece and the rules of the game, you can predict the next move with absolute certainty. This perspective can bring a sense of comfort, as it suggests a universe governed by order and predictability.
On the flip side, indeterminism introduces a level of uncertainty that can be both thrilling and perplexing. This viewpoint embraces the idea that not all events are predetermined; instead, some outcomes are genuinely random. Think of rolling a die: while the physical conditions at the moment of the roll influence the outcome, the exact result remains unpredictable. This randomness is essential in various scientific fields, particularly in quantum mechanics, where particles can exist in multiple states until measured. Here, the universe feels more like a chaotic dance than a rigid clock, inviting a myriad of possibilities.
The debate between these two perspectives raises fascinating questions: If everything is predetermined, what does that mean for free will? Can we truly say we make choices, or are we merely following a path laid out by the cosmos? Conversely, if indeterminism reigns, how do we reconcile the apparent order we observe in the natural world with the chaos of randomness? These inquiries not only impact philosophical discourse but also influence scientific methodologies and interpretations of probability.
To illustrate the contrast between determinism and indeterminism, consider the following table:
Aspect | Determinism | Indeterminism |
---|---|---|
Nature of Events | Pre-determined by prior states | Random and unpredictable |
Example | Chess game outcomes | Rolling a die |
Philosophical Implications | Challenges to free will | Possibility of multiple outcomes |
Ultimately, the discussion of determinism versus indeterminism is not just an academic exercise; it has real-world implications for how we understand probability in science. As researchers grapple with these concepts, they must consider how their philosophical beliefs shape their interpretations of data and the conclusions they draw. Whether one leans toward the certainty of determinism or the unpredictability of indeterminism, the interplay between these two philosophies continues to enrich our understanding of chance and probability in the scientific realm.
- What is determinism? Determinism is the philosophical belief that all events, including moral choices, are determined completely by previously existing causes.
- What is indeterminism? Indeterminism is the idea that not all events are determined by preceding events, allowing for randomness and unpredictability.
- How do these concepts affect scientific research? The belief in determinism or indeterminism can influence how scientists interpret data and the conclusions they draw from their experiments.
- Can free will exist in a deterministic universe? This is a widely debated question, with arguments on both sides regarding the nature of choice and responsibility.
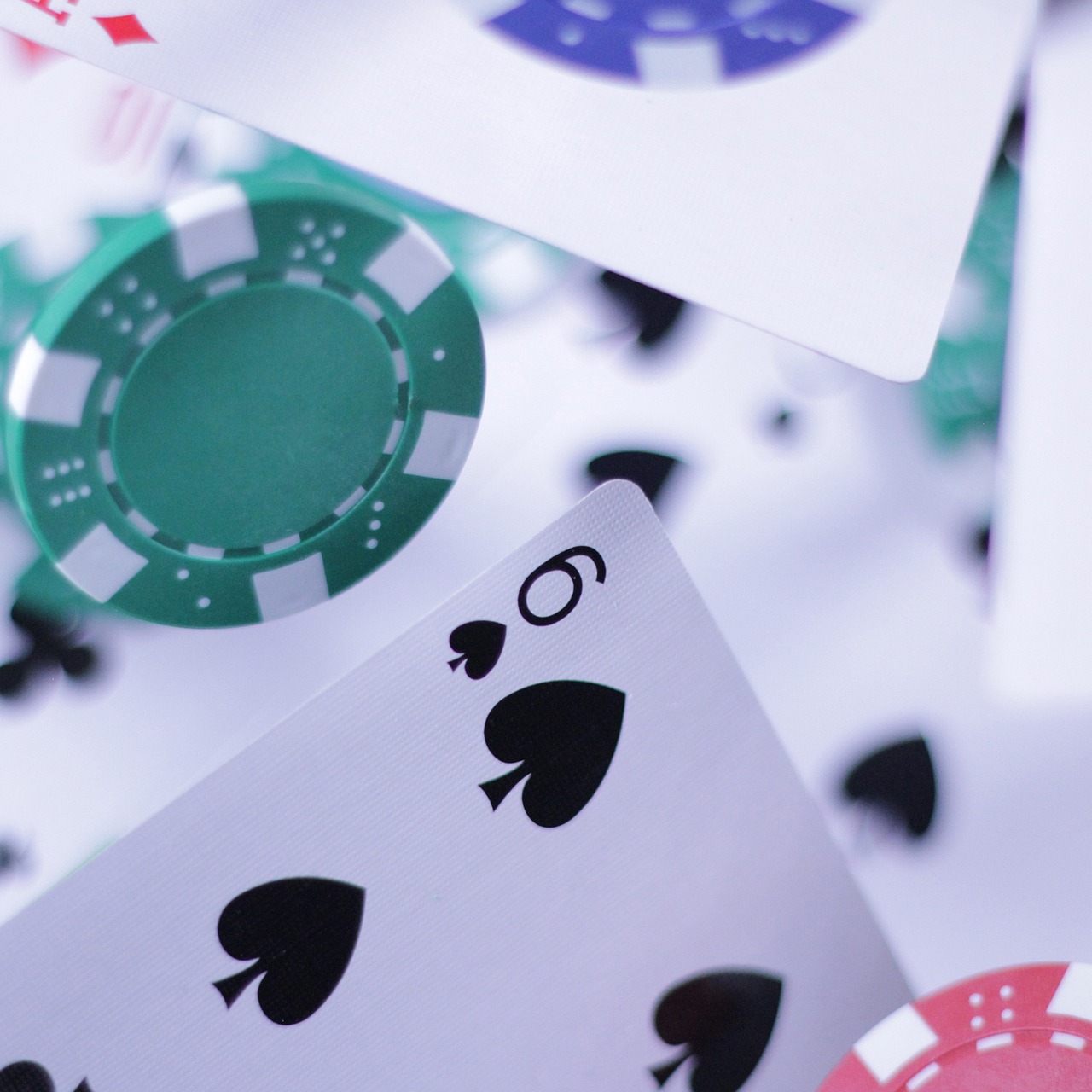
Subjective vs. Objective Probability
When we dive into the world of probability, we encounter two distinct yet interconnected perspectives: subjective and objective probability. Understanding the difference between these two approaches is crucial for interpreting chance and making informed decisions in scientific research. So, what exactly do these terms mean? Let's break it down.
Objective probability refers to the likelihood of an event occurring based on empirical evidence and statistical reasoning. This perspective relies heavily on data, experiments, and observable phenomena. For instance, if you flip a fair coin, the objective probability of landing heads is 50%. This is derived from the inherent properties of the coin and the mechanics of the flip. In scientific contexts, objective probability is often used to establish models and predict outcomes, providing a solid foundation for hypotheses and theories.
On the flip side, we have subjective probability, which is influenced by personal beliefs, experiences, and individual judgments. This type of probability is not necessarily grounded in hard data but rather reflects what someone believes about the likelihood of an event based on their unique perspective. For example, a seasoned investor might feel that a particular stock has a higher chance of rising based on their previous experiences and insights, even if the objective data suggests otherwise. This subjective view can be incredibly valuable in fields like psychology, economics, and decision-making, where human intuition plays a significant role.
To illustrate the difference more clearly, consider the following table:
Aspect | Objective Probability | Subjective Probability |
---|---|---|
Definition | Based on empirical data and statistical analysis | Based on personal beliefs and experiences |
Example | Probability of rolling a 3 on a fair die is 1/6 | Feeling that it will rain tomorrow based on past weather |
Application | Used in scientific research and statistical modeling | Used in personal decision-making and risk assessment |
Both subjective and objective probabilities have their merits and limitations. While objective probabilities provide a reliable framework for scientific inquiry, subjective probabilities can offer insights that data alone might miss. This interplay between the two perspectives can lead to richer analyses and more nuanced understandings of probability in various contexts.
As we navigate through scientific research and decision-making, recognizing when to apply each type of probability can significantly enhance our ability to interpret data and predict outcomes. By blending objective data with subjective insights, we can create a more comprehensive understanding of chance and its implications in our lives and the universe.
- What is the main difference between subjective and objective probability? Objective probability is based on empirical data, while subjective probability is influenced by personal beliefs and experiences.
- Can subjective probability be used in scientific research? Yes, subjective probability can provide valuable insights, particularly in fields where human intuition and experience play a crucial role.
- How can one improve their understanding of probability? Engaging with both statistical data and personal experiences can enhance one's grasp of probability concepts.
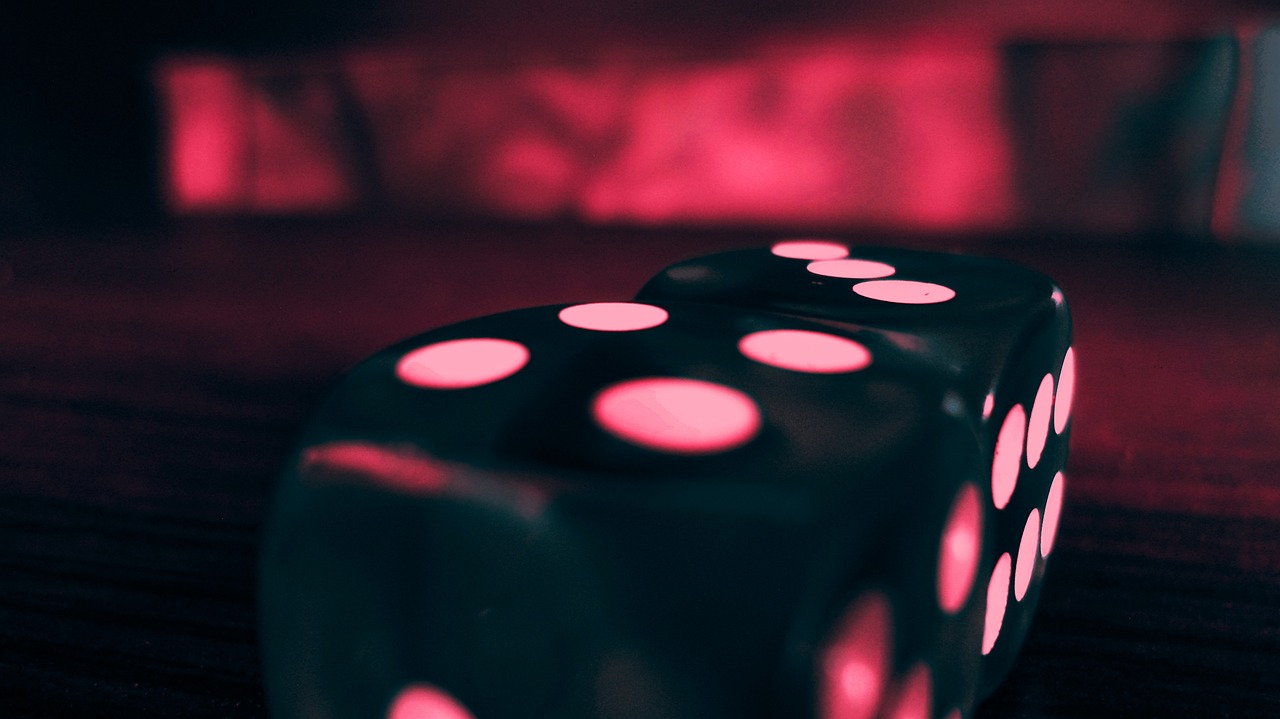
Applications of Probability in Science
Probability is not just a mathematical concept; it serves as the backbone of numerous scientific disciplines, influencing everything from the tiniest particles in quantum mechanics to the vast complexities of epidemiology. When we think about probability, we often picture dice rolls or lottery tickets, but in reality, its applications extend far beyond these simple examples. For instance, in the realm of quantum mechanics, probability is essential in predicting the behavior of particles at the subatomic level. Here, scientists often rely on probabilistic models to describe phenomena that are inherently uncertain. This uncertainty is not due to a lack of knowledge but rather a fundamental characteristic of the quantum world.
Moreover, in fields like epidemiology, probability plays a crucial role in understanding the spread of diseases. By analyzing patterns and using statistical models, researchers can predict how diseases will spread through populations, assess risks, and devise effective intervention strategies. For example, the infamous spread of the COVID-19 virus highlighted the importance of probability in public health decision-making. Scientists used probability models to estimate infection rates, project future outbreaks, and evaluate the effectiveness of various containment measures. These models often incorporate vast amounts of data, including transmission rates and population density, to create accurate predictions.
In addition to these fields, probability is also vital in engineering and computer science. In engineering, probabilistic methods are used to assess risks and ensure the reliability of structures and systems. For instance, when designing a bridge, engineers must consider the probability of various failure scenarios to ensure safety and durability. Similarly, in computer science, algorithms often rely on probabilistic models for tasks such as machine learning and artificial intelligence. By understanding and leveraging probability, these systems can make informed decisions based on uncertain data.
Field | Application of Probability |
---|---|
Quantum Mechanics | Predicting particle behavior and outcomes of experiments |
Epidemiology | Modeling disease spread and assessing public health risks |
Engineering | Risk assessment and reliability testing of structures |
Computer Science | Machine learning algorithms and data analysis |
Furthermore, the concept of probability is deeply integrated into the social sciences as well. Researchers use statistical methods to analyze data and draw conclusions about human behavior. For example, in psychology, probability helps in understanding the likelihood of certain behaviors occurring under specific conditions. This probabilistic approach allows scientists to make predictions about how individuals or groups will act, which can be incredibly valuable for developing interventions or policies.
In summary, the applications of probability in science are vast and varied, influencing numerous fields and helping researchers make sense of the complexities of the natural world. As we continue to explore the unknown, probability will undoubtedly remain a key tool in our scientific toolkit, guiding us through uncertainty and enabling us to make informed decisions based on the best available evidence.
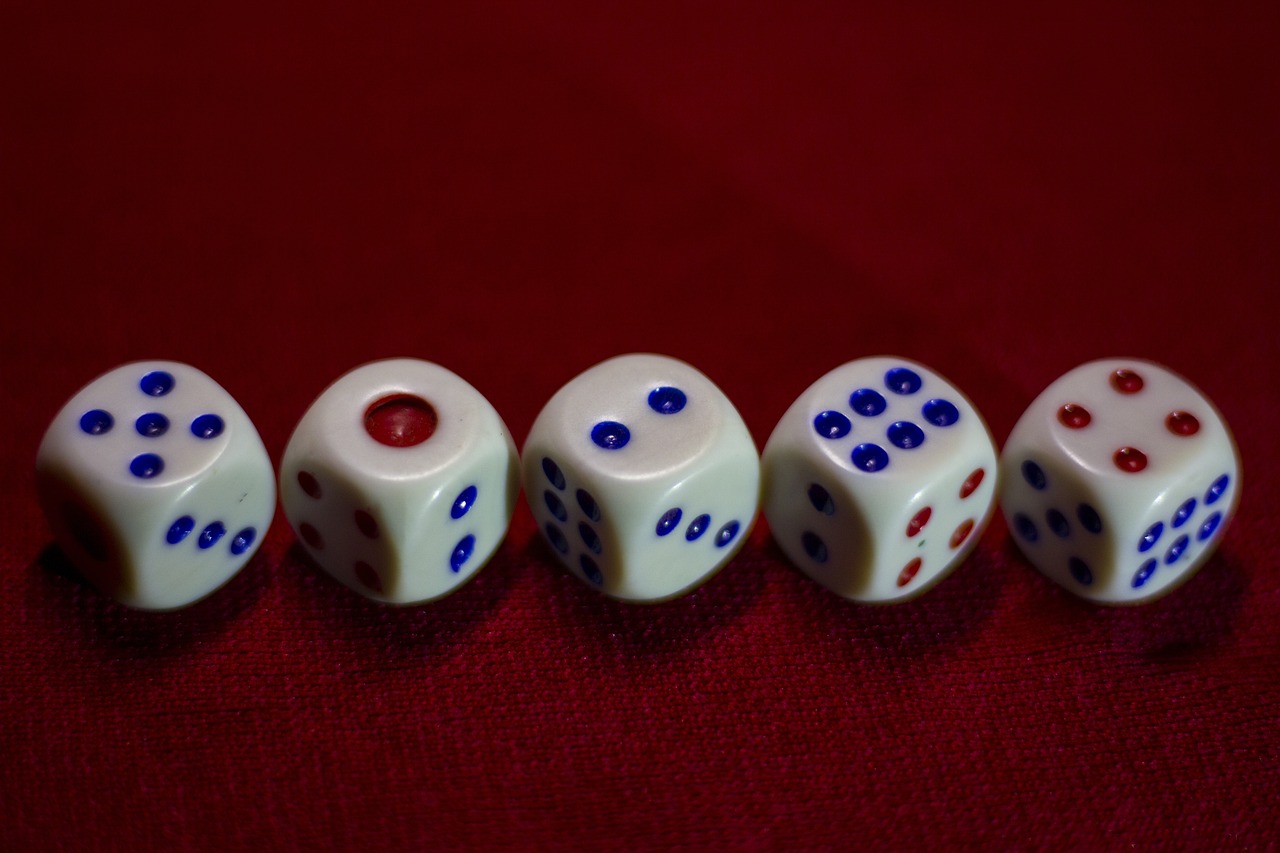
Challenges in Understanding Probability
Probability is a fascinating yet often misunderstood concept that plays a pivotal role in scientific inquiry. Despite its significance, many people encounter challenges when trying to grasp the nuances of probability. One of the primary issues stems from misconceptions about randomness. Many individuals believe that randomness implies a lack of order or predictability, which can lead to erroneous interpretations of probabilistic outcomes. For instance, when flipping a coin, the common belief is that after several heads, a tail is "due" to occur. This fallacy, known as the gambler's fallacy, showcases how our intuitions about chance can mislead us.
Another challenge lies in the complexity of probabilistic models. These models often require a solid understanding of both the mathematical frameworks involved and the philosophical implications they carry. For example, in statistical mechanics, the behavior of particles is described by probabilistic laws, which can seem counterintuitive to those accustomed to deterministic views of the universe. This complexity can create barriers for scientists and researchers, as they must navigate through intricate calculations and theoretical interpretations to apply probability effectively in their work.
Moreover, the distinction between subjective and objective probability adds another layer of difficulty. Subjective probability is based on personal belief or experience, while objective probability relies on empirical data and statistical analysis. This dichotomy can lead to confusion, especially in fields like psychology and economics, where personal biases play a significant role in decision-making. Understanding when to apply each type of probability is crucial for accurate scientific assessment.
To further illustrate the challenges faced in understanding probability, consider the following table that highlights some common misconceptions versus the realities of probability:
Common Misconception | Reality |
---|---|
Random events will balance out in the short term. | Randomness does not guarantee balance in short sequences; long-term trends may differ. |
Probabilities are absolute truths. | Probabilities are estimates based on available information and can change with new data. |
More data always leads to better predictions. | Quality of data is more important than quantity; poor data can skew results. |
In summary, the challenges in understanding probability are multifaceted, stemming from misconceptions about randomness, the complexity of probabilistic models, and the differences between subjective and objective interpretations. As we continue to explore the realms of science, it becomes increasingly essential to address these challenges, ensuring that both scientists and the general public can appreciate the true nature of probability and its implications for understanding the universe.
- What is the difference between probability and chance?
Probability is a quantitative measure of the likelihood of an event occurring, while chance refers more broadly to the occurrence of events without any specific measure. - Why is understanding probability important in science?
Understanding probability helps scientists make predictions, assess risks, and interpret data accurately, which is essential in fields ranging from medicine to environmental science. - How can I improve my understanding of probability?
Engaging with practical examples, studying statistical methods, and exploring philosophical discussions on randomness can enhance your grasp of probability.
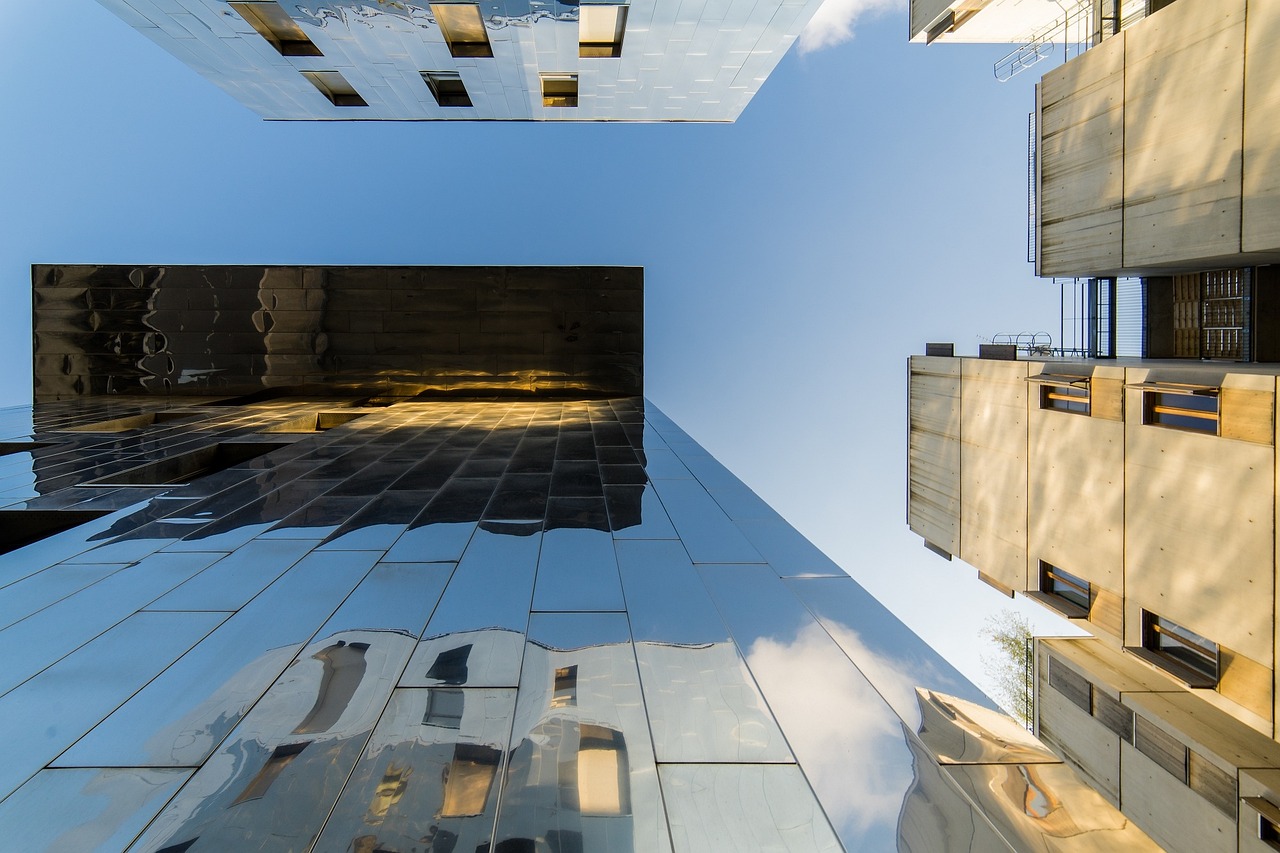
Common Misconceptions
When it comes to understanding probability, many people find themselves tangled in a web of misconceptions. These misunderstandings can lead to flawed reasoning and poor decision-making, especially in scientific contexts. One of the most prevalent misconceptions is the belief that probability is synonymous with randomness. While randomness is indeed a critical component of probability, they are not interchangeable terms. Probability is a measure of the likelihood of an event occurring, while randomness refers to the unpredictability of outcomes. Think of it this way: if you were to flip a coin, the probability of landing on heads is 50%. However, the actual outcome of each flip is random and can vary.
Another common misconception is the Gambler's Fallacy, which is the erroneous belief that past random events can influence future outcomes. For instance, if a coin has landed on heads several times in a row, one might assume that tails is "due" to occur. In reality, each coin flip is independent, and the probability remains the same at 50% for each flip. This fallacy can lead to poor betting strategies and misguided expectations in games of chance.
Moreover, many people struggle with the concept of independence in probability. They often think that if two events are independent, the occurrence of one must somehow affect the other. However, independence means that the outcome of one event does not influence the outcome of another. For example, rolling a die and flipping a coin are independent events; the result of the die roll has no bearing on whether the coin lands on heads or tails.
Additionally, the distinction between subjective and objective probability can be a source of confusion. Objective probability is based on empirical evidence and mathematical calculations, while subjective probability is shaped by personal beliefs and experiences. For instance, a scientist might use objective probability to predict the likelihood of a chemical reaction occurring based on previous experiments. In contrast, a person might feel that the chances of winning the lottery are higher based on their personal experiences, even though the mathematical odds remain the same.
To clarify these misconceptions, it’s essential to embrace a more nuanced understanding of probability. By recognizing the differences between these concepts, individuals can enhance their scientific reasoning and decision-making processes. Education and open discussions about probability can help demystify these common pitfalls, leading to a more informed perspective on how chance and probability interact in our lives.
- What is the difference between probability and randomness?
Probability measures how likely an event is to occur, while randomness refers to the unpredictable nature of outcomes. - What is the Gambler's Fallacy?
The Gambler's Fallacy is the mistaken belief that past independent random events can influence future outcomes. - How do subjective and objective probabilities differ?
Objective probability is based on mathematical calculations, while subjective probability is influenced by personal beliefs and experiences.
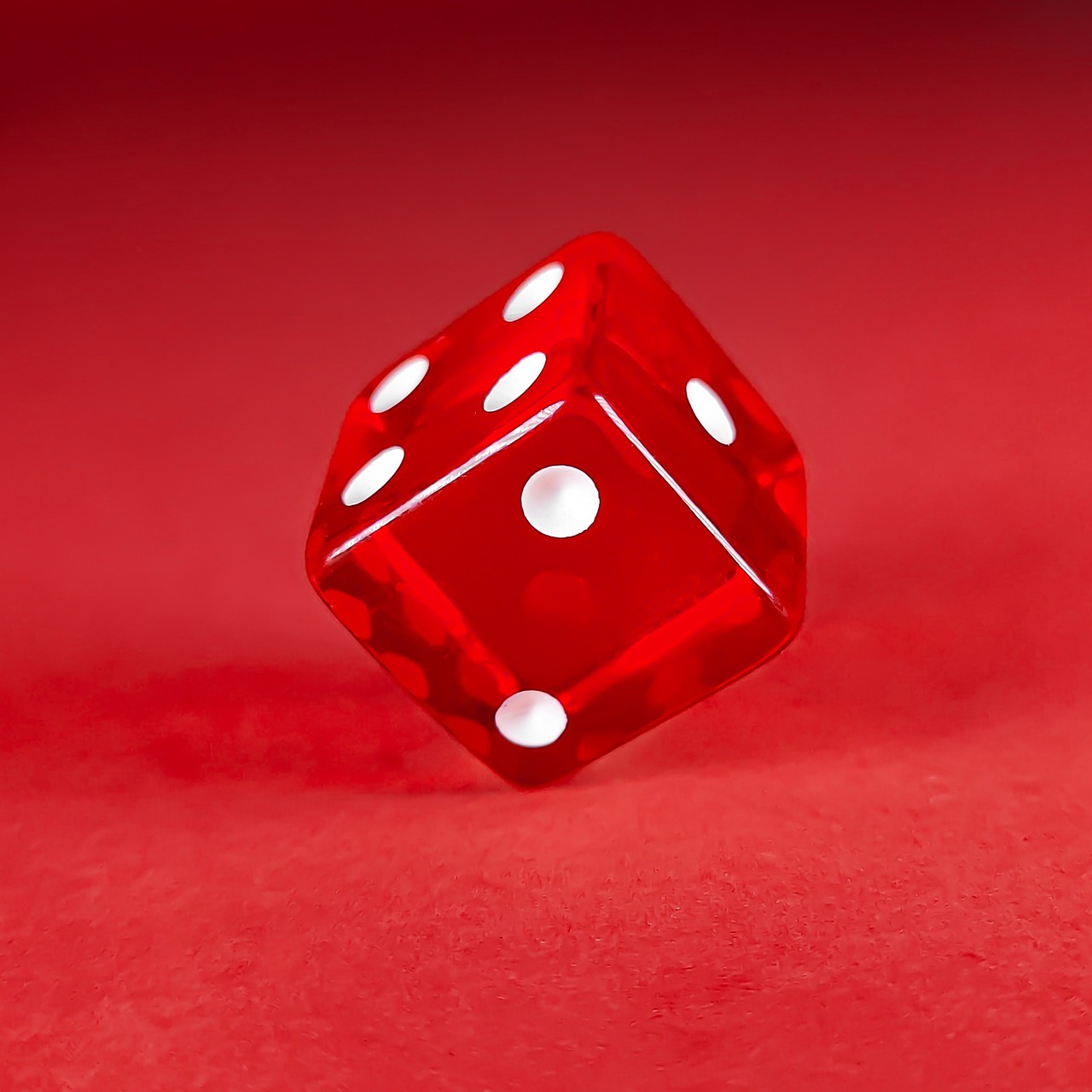
The Complexity of Probabilistic Models
When we dive into the world of probabilistic models, we often find ourselves in a labyrinth of complexity that can be both fascinating and daunting. These models serve as the backbone of many scientific inquiries, helping us to predict outcomes and understand phenomena that are inherently uncertain. However, the intricacies of these models can pose significant challenges for researchers and practitioners alike. Imagine trying to navigate a dense forest without a map; that’s what grappling with probabilistic models can feel like at times!
At their core, probabilistic models are designed to quantify uncertainty and manage the unpredictable nature of real-world events. They incorporate various statistical methods and mathematical theories to estimate probabilities, yet the sheer volume of variables and potential outcomes can make them overwhelming. For instance, consider a model used in epidemiology to predict the spread of a disease. It must account for numerous factors such as transmission rates, population density, and even social behavior, all of which can vary dramatically over time and across different locations.
Moreover, the complexity is not just in the number of variables but also in their interrelationships. These models often rely on assumptions that may not hold true in every situation, leading to potential errors in predictions. To illustrate, let’s take a look at a simple table that outlines some common types of probabilistic models and their complexities:
Model Type | Complexity Level | Common Applications |
---|---|---|
Bayesian Models | High | Medical diagnosis, machine learning |
Markov Models | Medium | Queueing theory, stock price predictions |
Monte Carlo Simulations | Very High | Risk assessment, financial forecasting |
As we can see, the complexity of these models varies, but they all share a common challenge: ensuring that the assumptions and inputs are accurate and relevant. This is particularly crucial in fields like finance or healthcare, where decisions based on flawed models can have serious consequences. So, how do scientists and researchers tackle these challenges? The answer lies in a combination of rigorous testing, validation, and a deep understanding of both the mathematical and philosophical underpinnings of probability.
Furthermore, the interpretation of the results generated by these models can also be complex. It’s not just about crunching numbers; it’s about understanding what those numbers mean in the context of the real world. For instance, a probabilistic model may yield a 70% chance of success for a new drug. But what does that really mean? Does it imply that the drug is guaranteed to work, or does it indicate a significant risk of failure? This ambiguity can lead to misunderstandings and misapplications in scientific research and public policy.
In conclusion, while probabilistic models are invaluable tools in scientific research, their complexity requires a nuanced approach. Researchers must not only be adept at the mathematical aspects but also possess a keen understanding of the philosophical implications of chance and uncertainty. As we continue to explore the relationship between chance and probability, it’s essential to remain vigilant about the challenges these models present and strive for clarity in both their construction and interpretation.
- What are probabilistic models?
Probabilistic models are mathematical frameworks used to represent and analyze uncertain events by estimating probabilities. - Why are probabilistic models important in science?
They help scientists make predictions and informed decisions in fields such as epidemiology, finance, and machine learning. - What challenges do researchers face with probabilistic models?
Challenges include understanding complex interrelationships between variables, ensuring accurate assumptions, and interpreting results correctly. - How can one improve their understanding of probabilistic models?
Engaging with statistical courses, practical applications, and discussions on philosophical implications can enhance understanding.
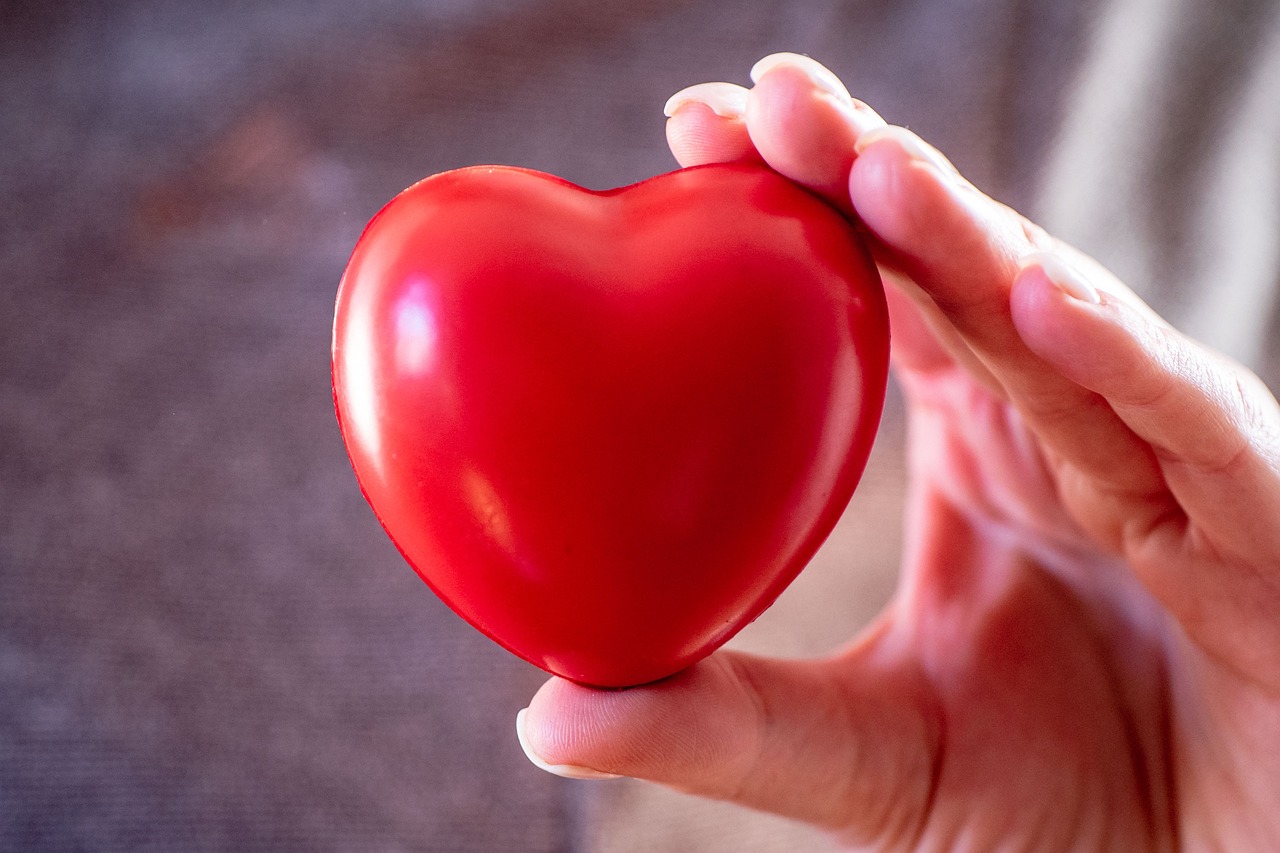
The Future of Probability in Science
The future of probability in science is not just a matter of numbers and equations; it’s a dynamic interplay between philosophy, mathematics, and real-world applications. As we continue to unravel the mysteries of the universe, the philosophical underpinnings of chance and probability will play a pivotal role in shaping our understanding of complex systems. Imagine standing on the edge of a vast ocean, where each wave represents a different outcome, and the currents symbolize the underlying probabilities that govern these outcomes. This metaphor captures the essence of how probability will guide future scientific endeavors.
One of the most exciting prospects is the integration of artificial intelligence (AI) with probabilistic models. As AI technologies advance, they will enable scientists to analyze vast datasets and identify patterns that were previously undetectable. For instance, in fields like genomics and climate science, AI can help predict outcomes with greater accuracy by leveraging probabilistic frameworks. This fusion of technology and probability could lead to breakthroughs that redefine our approach to scientific inquiry.
Moreover, the ongoing debate between determinism and indeterminism will continue to influence how we interpret probability. As we delve deeper into quantum mechanics, the inherent randomness of quantum events challenges traditional deterministic views. This philosophical shift may encourage scientists to embrace a more probabilistic mindset, acknowledging that uncertainty is an integral part of the natural world. Just as a dice roll introduces chance into a game, quantum events infuse unpredictability into the fabric of reality.
In addition, the importance of education in probability theory cannot be overstated. As future scientists are trained, a solid understanding of both the mathematical and philosophical aspects of probability will be essential. Educational institutions will need to adapt their curricula to emphasize the significance of probability in scientific reasoning. By fostering a culture that values probabilistic thinking, we can prepare the next generation of researchers to tackle complex challenges with confidence.
As we look ahead, several key areas will likely shape the future of probability in science:
- Interdisciplinary Collaboration: The blending of various scientific disciplines, such as biology, physics, and social sciences, will enhance our understanding of probabilistic models.
- Enhanced Computational Power: Advances in computing will allow for more sophisticated simulations and analyses of probabilistic scenarios, leading to more informed decision-making.
- Ethical Considerations: As probability plays a larger role in fields like healthcare and public policy, ethical implications will need to be carefully considered, ensuring that probabilistic models serve the greater good.
In conclusion, the future of probability in science is bright and full of potential. As we embrace uncertainty and the philosophical nuances of chance, we will unlock new pathways to understanding the universe. The journey ahead is not merely about predicting outcomes but also about appreciating the beauty of randomness and the intricate dance of probabilities that shape our reality.
- What is the significance of probability in scientific research? Probability helps scientists quantify uncertainty, making it easier to draw conclusions from data and predict future outcomes.
- How does AI influence the study of probability? AI enhances the ability to analyze large datasets, uncover patterns, and improve the accuracy of probabilistic models.
- What role does philosophy play in understanding probability? Philosophical perspectives shape our interpretation of randomness and chance, influencing how we apply probability in various scientific disciplines.
Frequently Asked Questions
- What is the relationship between chance and probability?
The relationship between chance and probability is intricate. Chance refers to the randomness of events, while probability quantifies the likelihood of those events occurring. In essence, probability provides a mathematical framework to understand and predict outcomes influenced by chance.
- How has the concept of chance evolved over time?
The concept of chance has evolved significantly from ancient philosophical views to contemporary scientific interpretations. Initially seen as a mystical force, it gradually transformed into a measurable entity that plays a crucial role in probability theory and scientific inquiry.
- What role does randomness play in scientific experiments?
Randomness is fundamental in scientific experiments as it ensures that results are not biased. By incorporating randomness, scientists can better understand the variability of outcomes, leading to more accurate interpretations of data and theories.
- What are the philosophical perspectives on randomness?
Various philosophical perspectives on randomness, such as determinism and indeterminism, offer different interpretations of how chance operates in the universe. These viewpoints influence how scientists understand and apply probability in their research.
- What is the difference between subjective and objective probability?
Subjective probability is based on personal judgment and beliefs about an event's likelihood, while objective probability relies on empirical data and statistical analysis. Understanding this distinction is crucial for accurate decision-making in scientific research.
- How is probability applied in different scientific fields?
Probability finds applications in various scientific fields, including quantum mechanics, epidemiology, and social sciences. Each field utilizes probability to model uncertainty, predict outcomes, and make informed decisions based on data analysis.
- What are some common misconceptions about probability?
Common misconceptions about probability include the belief that past events influence future outcomes (the gambler's fallacy) and misunderstanding the nature of independent events. Addressing these misconceptions is essential for enhancing the accuracy of scientific interpretations.
- Why are probabilistic models considered complex?
Probabilistic models can be complex due to their reliance on advanced mathematics and the philosophical implications of randomness. Understanding these models requires a deep comprehension of both the mathematical foundations and how they relate to real-world phenomena.
- What does the future hold for the philosophy of chance and probability in science?
The future of the philosophy of chance and probability in science is promising. As research continues to evolve, these concepts will play a vital role in shaping new discoveries and methodologies, prompting ongoing discussions about their significance in understanding the universe.